1. Abstract
A comparison of median rates of pay for the main groups of employees is reported in Annual Survey of Hours and Earnings - 2015 Provisional Results. These data provide comparisons for groups of employees but do not take into account the different composition and characteristics of these groups. This article presents the results of a statistical modelling approach which explores these compositional effects.
Section A provides an analysis of a range of factors affecting earnings that can be obtained from the Annual Survey of Hours and Earnings (ASHE). It does not include characteristics such as education which are not available in the ASHE dataset. The factors are used in a statistical regression model to explain hourly earnings, and are grouped by:
individual characteristics such as age and gender
job-related characteristics such as sector, occupation, region of employment, job tenure, job status and the size of organisation
The main results from the regression model are presented in Section B and are used to discuss the differences between public and private sector earnings, differences in earnings between men and women and by length of job tenure.
Nôl i'r tabl cynnwys2. Main points
Average pay levels such as those reported in the Annual Survey of Hours and Earnings - 2015 Provisional Results can vary between groups of employees because of the different jobs and characteristics of each type of employee. It is useful to compare like-with-like jobs and characteristics of employees in order to determine if any differences remain between earnings that can be attributed to the particular group
Public-private sector pay differences: In 2015, the average pay in the public sector was 3.5% less than in the private sector when using an established statistical model reported previously in Public and Private Sector Earnings - November 2014 which controls for individual and job-related characteristics, including organisational size
This pay gap is 0.2 percentage points smaller than in 2014, when average pay for the public sector was 3.7% less than the private sector
Repeating the analysis but excluding organisation size from the model, public sector employees earn 0.9% more than their private sector counterparts on average for April 2015
Age is a key variable in determining pay differences for different employees in the model as it can act as a proxy for work experience. It is also important to account for the differences in the age profile of employees by gender when considering differences in earnings between men and women
Gender pay differences: Using the same statistical model, at the median age of those employees observed in 2015 – 41 years old – men earned 13.5% more than women in 2015. This compares with a larger pay difference of 14.8% between the genders at age 41 years in 2014
Job tenure pay differences: the statistical model shows that average pay increases with job tenure. It is estimated that the average pay was between 3.0% and 25.5% higher for those with longer than 6 months job tenure, relative to those who had been in their job for less than 6 months in 2015, depending on length of tenure. This is broadly similar to the range of pay differentials by job tenure seen in 2014
3. Introduction
Headline measures of median earnings for employees in the UK are published in Annual Survey of Hours and Earnings - 2015 Provisional Results. Analysis presented in the bulletin covers median weekly earnings by age, gender, sector, region and occupation. Additional breakdowns are available for hourly and annual earnings. These data are useful for comparing groups of employees but do not take into account the different composition and characteristics of these employees. For example, comparing median earnings between employees in the public and private sectors, or between men and women does not take into account the additional factors that are common to these groups, such as the public sector having generally older workers than the private sector, or more women working in certain occupations compared with men.
This article addresses this issue and presents the results of a regression model, developed in 2011 by ONS and HM Treasury, which statistically controls for a range of factors related to earnings that are available in the ASHE dataset. These variables are listed in full in Section B. This enables the influence of separate factors on hourly earnings to be identified, such as working in the public or private sectors, while keeping other predictive factors constant.
The factors which are used in the regression model to explain hourly earnings can be grouped by:
individual characteristics such as age and gender
job-related characteristics such as sector, occupation, region of employment, job tenure, job status and size of employer
Section A of this article describes the factors which are used in the earnings regression model using the provisional ASHE dataset for April 2015. There are a number of important points to note about this analysis and the approach used in the regression model:
the ASHE dataset only covers the earnings of paid employees in the UK, and does not include data on self-employed earnings
hourly earnings, excluding overtime are used in this article, so that differences between the amounts of hours worked per week by different people are controlled for. An adjustment is made to hourly earnings (the dependent variable) in the ASHE dataset to better account for the timing of bonus payments throughout the year. Estimates in this article are not therefore directly comparable with those published in ASHE Provisional Results 2015 which do not make this adjustment for the timing of bonus payments
pension contributions by employers are not yet available in the 2015 ASHE dataset and the regression model has not been designed to take them into account
for April 2015 the data has been split by public and private sectors, where the private sector excludes employees in the Non Profit Institutions serving Households (NPISH). In the UK the NPISH sector includes organisations such as charities, trade unions and most universities. In some other surveys this group is included in the analysis of private sector organisations and employees. This treatment, however, is consistent with previous analysis of ASHE data where it was not possible to identify NPISH employees separately
Section B presents the key results from the regression model and discusses the differences between public and private sector earnings, differences in earnings between men and women and by length of job tenure. The background notes section provides details of how the presentation of this article differs compared to last year’s release.
Nôl i'r tabl cynnwys4. Section A: Factors affecting earnings
There are a range of factors affecting earnings which can be analysed using the ASHE dataset, which can be grouped by:
individual characteristics such as age and gender
job-related characteristics such as public or private sector, occupational group, region of employment, job tenure, job status and size of employer
Individual characteristics
Age is a key factor affecting earnings, as this tends to be a proxy for experience and the build-up of skills over time. Figure 1 shows that the hourly pay of all employees – regardless of sector - rises sharply between the ages of 17 and 42 years old, as job-related skills and experience is rewarded. Average pay rates remain at around the £18 per hour level for each year of additional age, until they begin to decline to between £14 and £16 per hour for older employees in the private and public sectors respectively.
Figure 1, although not accounting for the different jobs within each sector also shows that average hourly earnings are higher for younger workers in the public sector – relative to those in the private sector – although wage levels reach similar levels for both sectors by the late 30s. The private sector wage level is higher between the ages of 38 and 56, but declines faster after this age compared with the public sector.
Figure 1: Mean hourly earnings for all employees by age and sector, April 2015
UK
Source: Annual Survey of Hours and Earnings (ASHE) - Office for National Statistics
Notes:
- Data for 2015 is provisional
- The hourly pay of all employees rises sharply between the ages of 17 and 42 years old, as job related skills and experience is rewarded
- The private sector wage level is higher between the ages of 38 and 56, but declines faster after this age compared with the public sector
Download this chart Figure 1: Mean hourly earnings for all employees by age and sector, April 2015
Image .csv .xlsThe age profile of workers also varies significantly by whether they are employed in the public or private sector. Figure 2 shows that the distribution of jobs held within the private sector is more skewed towards younger age groups, while the distribution of jobs held within the public sector is more skewed towards older age groups.
Figure 2: Age profile by Public and Private sector, April 2015
UK
Source: Annual Survey of Hours and Earnings (ASHE) - Office for National Statistics
Notes:
- Data for 2015 is provisional
- The distribution of jobs held within the private sector is more skewed towards younger age groups
- The distribution of jobs held within the public sector are more skewed towards older age groups
Download this chart Figure 2: Age profile by Public and Private sector, April 2015
Image .csv .xlsA similar analysis of the distribution of jobs held by men and women at each age in Figure 3 shows that the employment share of females drops by around half a percentage point between the ages of around 26 years to around 38 years, and then starts to increase from age 40 years onwards until age 50 years. The employment share of men and women then decreases from each year of age at similar rates.
Figure 3: Age profile by gender, April 2015
UK
Source: Annual Survey of Hours and Earnings (ASHE) - Office for National Statistics
Notes:
- Data for 2015 is provisional
- The employment share of females drops by around half a percentage point from around the age 26 years at each age, and then starts to increase from age 40 years onwards until age 50 years
- The employment share of men and women then decreases from each year of age at similar rates
Download this chart Figure 3: Age profile by gender, April 2015
Image .csv .xlsJob-related characteristics
Public or Private Sector
The regression analysis in this paper allows us to identify the differences in hourly earnings between the public and private sectors when a variety of other job and personal characteristics are held constant. As already highlighted, there are differences between the sectors related to the age of the employees. There are also differences between the sectors when split by gender.
Figure 4 shows how the share of employee jobs within each sector differs by gender, with women making up over two-thirds of public sector employees compared with less than half of employees in the private sector.
Figure 4: Gender share of employee jobs by sector, April 2015
UK
Source: Annual Survey of Hours and Earnings (ASHE) - Office for National Statistics
Notes:
- Data for 2015 is provisional
- The share of employee jobs within each sector differs by gender, with women making up two thirds of public sector employees
- Compared with less than half of employee jobs in the private sector
Download this chart Figure 4: Gender share of employee jobs by sector, April 2015
Image .csv .xlsDistribution of earnings by sector
Figure 5 shows that the earnings of the public sector are generally higher for all employees across the earnings distribution, apart from the top 10% of earners. In April 2015 – for employees in the 99th percentile of employees by hourly earnings – the private sector earned around £20 per hour more than those employees in the public sector.
Figure 5: Distribution of hourly earnings in the public and private sector, April 2015
UK
Source: Annual Survey of Hours and Earnings (ASHE) - Office for National Statistics
Notes:
- Data for 2015 id provisional
- The earnings of the public sector are generally higher for all employees across the earnings distribution
- Apart form the top 10% of earners (those above the 90th percentile)
- For employees at the 99th percentile those in private sector earned £20 per hour more than in the public sector
Download this chart Figure 5: Distribution of hourly earnings in the public and private sector, April 2015
Image .csv .xlsRegion
Figure 6 shows that there are clear differences in average hourly pay across the regions of work, particularly in relation to London and the South East reflecting the nature of work in these areas and other factors likely to be associated with regions such as the costs of living. Table 1 illustrates the differences in the share of occupations in each region and country of the UK.
Figure 6: Mean hourly earnings by region of England and devolved administrations of the UK, £ per hour (all employees), April 2015
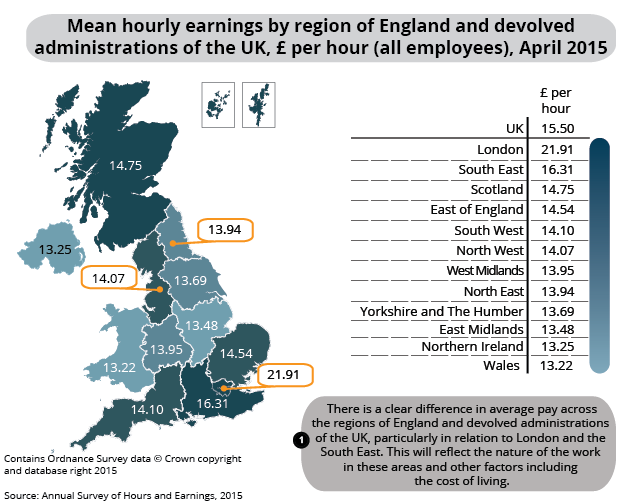
Source: Annual Survey of Hours and Earnings (ASHE) - Office for National Statistics
Notes:
- Data for 2015 is provisional
Download this image Figure 6: Mean hourly earnings by region of England and devolved administrations of the UK, £ per hour (all employees), April 2015
.png (79.1 kB) .xls (26.6 kB)Table 1 shows the shares of employment for the professional occupations – which tend to be paid a higher wage due to the skill level of the job – compared with elementary occupations (using the Standard of Occupational Classification (SOC 2010)) in each region and devolved administration of the UK. Professional occupations include scientists, IT engineers, health and educational professionals, while elementary occupations include farm workers, window cleaners, waiters, waitresses and processing operatives.
We see that, in April 2015, 25.8% of employee jobs in London were classified as professional occupations. By comparison, in the East Midlands, professional occupations accounted for 16.7% of employee jobs. Comparing the regions' share of elementary jobs, 15.4% of all employees in the East Midlands – and 8.6% of all employees in London – were classified as elementary.
Table 1: Share of professional and elementary occupational groups by regions of England and devolved nations, April 2015
% | ||
Geographic Breakdown | Professional occupations (SOC 2100–2499) | Elementary occupations (SOC 9100-9299) |
North East | 19.7 | 11.8 |
North West | 19.8 | 12.4 |
Yorkshire and The Humber | 18.6 | 13.6 |
East Midlands | 16.7 | 15.4 |
West Midlands | 18.6 | 12.9 |
South West | 19.5 | 12.6 |
East | 20.3 | 12.4 |
London | 25.8 | 8.6 |
South East | 22.5 | 10.6 |
Wales | 19.8 | 12.8 |
Scotland | 22.2 | 11.0 |
Northern Ireland | 21.3 | 10.9 |
Source: ONS Annual Survey of Hours and Earnings | ||
Notes: | ||
1. Data for 2015 is provisional |
Download this table Table 1: Share of professional and elementary occupational groups by regions of England and devolved nations, April 2015
.xls (26.1 kB)Tenure: The length of time an individual has been working in their current job can also add to their earnings as employers recognise job-specific skills that have been built up through experience in a role. Table 2 shows how the hourly wage increases with length of tenure ranging from around £10.90 per hour for tenures of 6 months or less, to just over £20 per hour for tenures of 20 years or more.
Table 2: Mean hourly earnings by job tenure, April 2015, UK
Length of Job Tenure | £/ hour |
6 months or less | 10.91 |
Between 6 months and 1 year | 12.37 |
Between 1 and 2 years | 13.44 |
Between 2 and 5 years | 15.33 |
Between 5 and 10 years | 16.58 |
Between 10 and 20 years | 18.23 |
Over 20 years | 20.12 |
Source: ONS Annual Survey of Hours and Earnings | |
Notes: | |
1. Data for 2015 is provisional |
Download this table Table 2: Mean hourly earnings by job tenure, April 2015, UK
.xls (32.3 kB)In April 2015, Figure 7 shows that there is much greater share of employees with longer tenures within the public sector compared to the private sector. For example, around 40% of public sector workers have tenures of over 10 years, and 14% of public sector workers in April 2015 have tenures of over 20 years. This compares with around 23% of private sector workers who have tenures of more than 10 years and just over 7% of private sector employees who have tenures of over 20 years.
Figure 7: Share of employee jobs by sector and by length of tenure, April 2015
UK
Source: Annual Survey of Hours and Earnings (ASHE) - Office for National Statistics
Notes:
- Data for 2015 is provisional
- There is a greater share of employees with longer tenures within the public sector compared with the private sector
- Around 40% of public sector employees have tenures of over 10 years, compared with 23% of private sector employees
Download this chart Figure 7: Share of employee jobs by sector and by length of tenure, April 2015
Image .csv .xlsJob status: Full-time or part-time, temporary or permanent: There are differences in hourly earnings when comparing types of jobs e.g. whether they are full-time or part-time, or whether they are permanent or temporary.
Table 3 shows that mean hourly earnings of full-time employees was around £5.50 higher than for part-time employees, and a slightly lower differential, of around £3.30 per hour, was seen between employees with permanent jobs compared with temporary jobs.
Table 3 also shows that the share of full-time jobs is 5 percentage points higher in the private sector compared to the public sector, and the share of permanent jobs is 3 percentage points higher in the private sector.
Table 3: Mean hourly earnings by job characteristic, and share of jobs by sector, April 2015, UK
Characteristic | Earnings (£/ hour) | Share of employee jobs in public sector (%) | Share of employee jobs in private sector (%) |
Full-time | 17.09 | 69 | 74 |
Part-time | 11.46 | 31 | 26 |
Permanent | 15.78 | 90 | 93 |
Temporary | 12.36 | 10 | 7 |
Source: ONS Annual Survey of Hours and Earnings | |||
Notes: | |||
1. Data for 2015 is provisional |
Download this table Table 3: Mean hourly earnings by job characteristic, and share of jobs by sector, April 2015, UK
.xls (54.3 kB)Organisational size: The size of the employer organisation can influence rates of pay, with the potential that larger employers may be able to pay higher wages for similar roles than smaller ones where profit margins may be narrower. The regression analysis in this article breaks down the size of employer into 6 bands, the bands relating to the number of employees in the organisation.
Figure 8: Percentage of employee jobs by size of organisation and by sector, April 2015
UK
Source: Annual Survey of Hours and Earnings (ASHE) - Office for National Statistics
Notes:
- Data for 2015 is provisional
- In April 2015, 57.3% of all employee jobs were in large organisations over 500 employees
- There are differences between the public and private sectors. 90% of public sector employees work in these large organisations, compared with 46.5% of private sector workers
- Around 12% of private sector employees work in organisations with fewer then 11 employees compared with 0.2% of public sector employees
Download this chart Figure 8: Percentage of employee jobs by size of organisation and by sector, April 2015
Image .csv .xlsFigure 8 shows that in April 2015, 57.3% of all employee jobs (which includes NPISH sector employees and those unclassified by sector) were in large organisations (over 500 employees), with differences between the public and private sectors. Around 90% of public sector employees are employed in organisations of more than 500 employees which include organisations such as the NHS, fire and police services or local authorities. In contrast, less than half of those employed in the private sector worked in large organisations.
At the other end of the organisational size range, around 12% of private sector employees worked in organisations of 10 employees or fewer, whereas 0.2% of public sector employees work for this type of small organisation.
Nôl i'r tabl cynnwys5. Section B: Regression Analysis for the UK
The following regression analysis estimates pay difference between types of individuals when controlling for those factors discussed in Section A and listed below.
The primary source of data is the Annual Survey of Hours and Earnings (ASHE) using hourly earnings (excluding overtime) for all employees whose pay in the April period was not affected by absence and were paid adult rates. This data has been adjusted to account for bonus payments paid outside of the period covered by ASHE using data from the Average Weekly Earnings (AWE) series.
The multiple linear regression model used is specified as follows:
Dependent Variable:
- log of bonus adjusted hourly earnings excluding overtime (see Statistical Notes 1 and 4)
Independent Variables for “Individual Characteristics”:
gender - because of differences in the distribution of men and women. This is a dummy variable showing men relative to women
age - because the relationship between earnings and age is non-linear. Due to the filters of the data, the youngest an employee can be is 16 years old, therefore, estimates are relative to this
age Squared (See Statistical Note 2)
Independent Variables for “Job-related characteristics”:
employment status – full- and part-time employment – because full-time workers tend to be paid more per hour than part-time workers
employment status – Permanent or temporary employment – because permanent workers tend to be paid more on average than temporary workers
job Tenure based on days worked in the employee’s current job – less or equal to 6 months, 6 to 12 months, 1 year to 2 years, 2 to 5 years, 5 to 10 years, 10 to 20 years, and over 20 years. Job tenure is a proxy for organisation-specific experience
sector (public or private)
occupations (coded to SOC 2010 for 2011-2013 and coded to a SOC 2010 equivalent for 2002-2010) - Because pay is influenced by occupation
region (that the job is located in – 12 across the UK) - because of differences in the nature of employment across the country
organisation size, categorised into six bands, by the number of employees in the associated firm: Less than or equal to 10, 11-25, 26-50, 51-250, 251-500, 501+
Interaction Terms (See Statistical Note 3):
gender*Age and Gender*Age squared - the potential work experience proxies by age for males and females are different, i.e. women experience more career interruptions than males
occupation*Region – Industry and labour market structures that impact on wages may differ between regions
organisation size*Occupation - Because evidence shows large organisations tend to pay more than small organisations for the same job
occupation*Age – The return to work experience may be different for different occupations
occupation*Age Squared – The return to work experience may be different for different occupations
Analysis of key factors
Public/private sectors
Comparing sectors and controlling for the variables set out above (including organisation size); on average public sector employees in the UK earned 3.5% less than private sector employees in 2015, as shown in Figure 9. This implies a slight narrowing of the pay differential over the last year, with public sector employees earning around 3.7% less than their private sector counterparts in 2014 – although, as Figure 9 shows, the series is somewhat volatile.
The model results show that in controlling for the factors in Section A provide the opposite result to the comparison of median rates of hourly earnings where the public sector is paid more on average than the private sector.
Figure 9: Average difference in mean hourly pay (excluding overtime) between public and private sector workers expressed as a percentage of private pay, April 2002 to April 2015
UK
Source: Annual Survey of Hours and Earnings (ASHE) - Office for National Statistics
Notes:
- No data is available for Northern Ireland for 2002 to 2003, estimates for these years refer to Great Britain
- Data for 2015 is provisional
- On average public sector employees in the UK earned 3.5% less than private sector employees in 2015
- The pay differential has narrowed slightly from 3.7% in 2014
Download this chart Figure 9: Average difference in mean hourly pay (excluding overtime) between public and private sector workers expressed as a percentage of private pay, April 2002 to April 2015
Image .csv .xlsPrevious analysis in the Public and Private Sector Earnings - November 2014 also observed the pay differentials between sectors, using the same model, but excluding the impact of organisation size. This was done for a number of reasons.
Firstly, if the view is held that public sector employees should earn the same as private sector employees irrespective of organisation size, then it would be useful to see the results without organisation size included.
From a statistical point of view, as over 90% of those working in the public sector are also in large organisations – as shown in Figure 8 – the inclusion of organisation size can lead to issues of collinearity and have an impact on the precision of the estimate of the public/private sector pay differential. The model with organisational size however still remains statistically valid.
Repeating the analysis but excluding organisation size from the model, public sector employees earn 0.9% more than their private sector counterparts on average for April 2015. This compares to a 0.6% differential in April 2014. It is implied in both cases – with and without organisational size included in the regression model – that growth in mean hourly earnings for employees in the public sector has grown slightly faster (all other factors being equal) than in the private sector between 2014 and 2015.
The Reference Table (263 Kb Excel sheet) has more details of the regression results for public and private sector pay, excluding organisational size.
Sector and region
When expanding the model to control for interactions between sectors and regions, Figure 10 shows that the sector pay differential in a number of regions and devolved administrations of the UK is smaller than the UK average and the relationship reversed in others. However, for London and the South East there is a statistically significant larger pay differential (public sector paid less than private sector) than the UK average in 2015.
That being said, Figure 10 also shows 95% confidence intervals. These illustrate an estimated range of values that the true value, with a 95% certainty, lies within. They suggest that, while these estimates differ from the UK Average, for those regions with overlapping confidence intervals, the pay gap in each region may not be statistically significantly different from each other.
Figure 10: Average difference in mean hourly pay (excluding overtime) between public and private sector employees regions of England and the devolved administrations of the UK
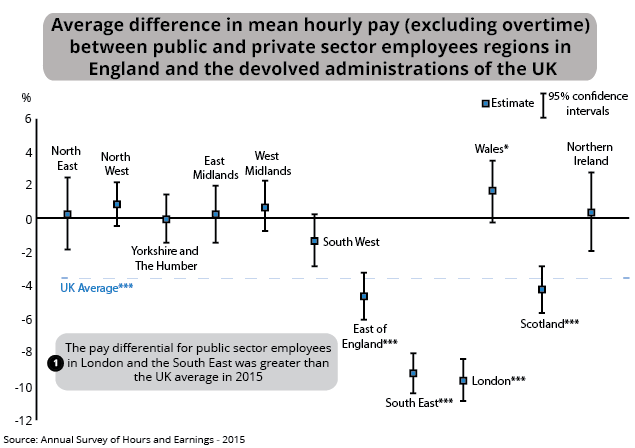
Source: Annual Survey of Hours and Earnings (ASHE) - Office for National Statistics
Notes:
- Data for 2015 is provisional
- This percentage is the difference between private and public sector pay as a percentage of private sector pay
- 95% confidence intervals for the estimates
- Model used accounts for organisation size
- *** p<0.01, ** p<0.05, * p<0.1
Download this image Figure 10: Average difference in mean hourly pay (excluding overtime) between public and private sector employees regions of England and the devolved administrations of the UK
.png (35.9 kB) .xls (28.2 kB)Age and Gender
The age of an employee, similarly, has a positive impact on earnings as – for each additional birthday – women earn on average an extra 1.9%, while men earn on average 3% more for each year. However, the relationship between age and earnings appears to be non-linear (See Statistical Note 2), suggesting that while it may be positive and significant in the initial years, the effect of age on earning lessens as the individual gets older.
Gender is somewhat more complicated to interpret from this model. This is because not only do we have a “gender” dummy variable – where female=0 and male=1 – but we also have interaction terms that take gender into account, in particular the interaction between gender and age. An explanatory note is included in the Statistical Notes to this section (See Statistical Note 3). As a result the pay differential between men and women changes throughout a lifetime, as is shown in Figure 11.
However, at the median age of those employees observed in 2015 – 41 years old and shown by the vertical dotted black line – men on average were paid 13.5% more than women. This compares to 41 year old men earning 14.8% more on average than women of the same age in 2014.
Figure 11: Average difference in mean hourly pay (excluding overtime) between men and women, aged 16 to 64, April 2015, UK
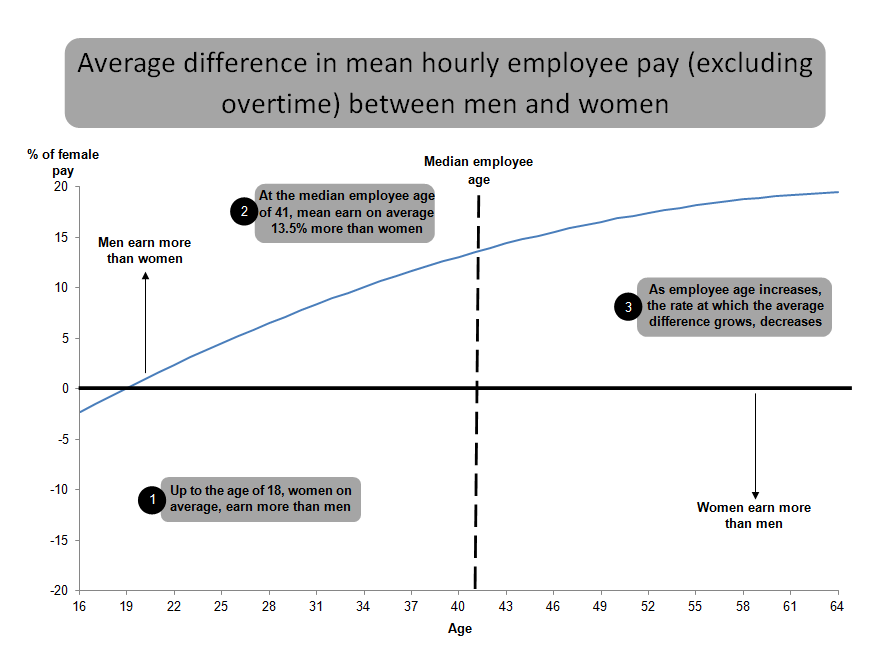
Source: Annual Survey of Hours and Earnings (ASHE) - Office for National Statistics
Notes:
- Data for 2015 is provisional
- This percentage is the difference between male and female pay as a percentage of female pay
Download this image Figure 11: Average difference in mean hourly pay (excluding overtime) between men and women, aged 16 to 64, April 2015, UK
.png (37.5 kB) .xls (28.7 kB)Job Tenure
One of the largest impacts on earnings, with all other factors being equal, comes from an employee’s job tenure – how long an employee has been in their current job. Figure 12 compares the pay differential of the job tenure groups to those who have been in their job for half a year or less and suggests that the longer a person is in a job, the more they earn, allowing for all other individual and job-related factors such as gender, occupation and region.
At the same time the model suggests this relationship holds with each subsequent group earning more than the previous group, up to those in the same job for 20 years or more earning 25.5% more than those newly started in a job.
Figure 12: Average difference in mean hourly pay (excluding overtime) by job tenure group, aged 16 to 64, 2015, UK
Source: Annual Survey of Hours and Earnings (ASHE) - Office for National Statistics
Notes:
- Data for 2015 is provisional
- This percentage is the difference in pay compared with the reference group. The reference group is those that have been employed for less than 6 months
- These percentage are the pay differences for employees of the various job tenures below compared with an employee whose job tenure is less than 6 months
- Compared to a job tenure of less than 6 months, the longer an employee is in a job the more they earn
- Those that have been in their job for more than 20 years earn, on average, 25.5% more than those with a job tenure of less than 6 months
Download this chart Figure 12: Average difference in mean hourly pay (excluding overtime) by job tenure group, aged 16 to 64, 2015, UK
Image .csv .xlsAnalysis of other factors
Part-time/full-time status; permanent/temporary status
While unable to observe those self-employed, we can see that employees either in part-time work or working temporarily have lower average earnings relative to their counter statuses. When comparing over the last year, the estimates for 2015 suggest both pay differentials – part-time work and working temporarily – have increased since 2014 from -7.1% to -8.1% and 0.0% to -1.9% respectively.
Occupation and region
When looking at “job-related characteristics” such as occupation or region, the interpretations become a little more complex. This is because when interpreting the model’s “job-related characteristics”, we have to be aware of any associated interaction terms (See Statistical Note 3) and we are able to report pay differentials given a number of controls.
So, when comparing those in elementary occupations across the UK regions in 2015, the model also suggests that working outside London has a negative impact on earnings. The majority of the regions earned between 2.8% to 9.0% less on average than those in the capital with the equivalent job. Only the South East and Scotland were not statistically different from zero.
Observing for different occupations, the model suggests that the relationship holds with the regions outside of London experiencing relatively lower average pay. This ranges from Skilled Trades Occupations in Northern Ireland earning 6% less on average in 2015 than those Skilled Trades Occupations in London, and Chief Executives and Senior Officials in the North West earning 63.9% relatively less on average in the same year compared to London.
Similar to when we observed those in elementary occupations by region, in switching between different occupation groups, a number of combinations are not statistically different to London. The interpretation, however, of these should take into account whether they are truly not significant or whether it is as a result of small sample size. This is an issue to be expected when looking so granularly.
Organisational size
We can similarly observe the effect of how large a firm someone is employed by. Figure 13 shows impact of organisational size on employees’ earnings, relative to employees in organisations with more than 500 employees and in elementary occupations.
Figure 13: Average difference in mean hourly pay (excluding overtime) by organisation size, aged 16 to 64, 2014 and 2015, UK
Elementary Occupation only
Source: Annual Survey of Hours and Earnings (ASHE) - Office for National Statistics
Notes:
- Data for 2015 is provisional
- This percentage is the difference in pay compared with the reference group. The reference group is those organisations with more than 500 employees and in Elementary Occupation group
- Compared to those employees in an organisation with over 500 employees
- All employees in other organisation sizes earn less on average
- The size of this difference has increased from 2014 to 2015
Download this chart Figure 13: Average difference in mean hourly pay (excluding overtime) by organisation size, aged 16 to 64, 2014 and 2015, UK
Image .csv .xlsIt suggests that those employed in all breakdowns of smaller firms earn relatively less on average, with the difference in pay for all groupings increasing, relative to large firms, between 2014 and 2015. What Figure 13 also shows is that the pay differentials are not as regressive as other similar style variables observed, with the impact being much more uniform amongst firms of differing size.
Statistical Notes:
The dependent variable is expressed in log form. If the distribution of a variable has a positive skew, taking a natural logarithm of the variable sometimes helps fitting the variable into a model. Also, when a change in the dependent variable is related with percentage change in an independent variable, or vice versa, the relationship is better modelled by taking the natural log of either or both of the variables.
When accounting for the age of employees in the regression model, we have incorporated a variable for both Age and Age Squared; this is due to the Taylor series approximations. Taylor series approximations tell us that for many smooth functions, they can be approximated by a polynomial, so including terms like χ2 or χ3 let us estimate the coefficients for the approximation for a known or unknown non-linear function of χ, or in this case age.
As well as the suite of independent variables observed in the model, a number of interaction terms are included. These are added to account for the assumption that some characteristics interact with one another.
The presence of a significant interaction indicates that the effect of the 1st independent variable (α1) on the dependent variable (Ω) is different at different values of a 2nd independent variable (α2). It is tested by adding a term to the model in which the two independent variables are multiplied.
Ω= A + B1*α1 + B2*α2+ B3*α1*α2
Adding an interaction term to a model drastically changes the interpretation of all of the coefficients. If there were no interaction term, B1 would be interpreted as the unique effect on the Ω (in this case pay). But the interaction means that the effect of α1on Ω is different for different values of α2. So the unique effect of α1 is not limited to B1, but also depends on the values of B3 and α2.
The unique effect of α1 is represented by everything that is multiplied by α1in the model: B1 + B3* α2. B1 is now interpreted as the unique effect of α1 on Ω only when α2= 0.
When interpreting the outputs of the model, care needs to be taken with the coefficients of variables. While the independent variables are in their original state, the dependent variable is in its log-transformed state, therefore, the coefficient (β) for the independent variables do not simply reflect the percentage change but (100*β) percent for a one unit increase in the independent variable, with all other variable in the model held constant.