Cynnwys
- Introduction
- Acknowledgements
- Disclaimer
- Design of the admin-based population estimates and their statistical uncertainty
- Comparison of ABPEs with official population estimates time series
- What can we learn from statistical uncertainty in the ABPEs?
- What can we learn from comparing 2011 ABPE and mid-year estimates uncertainty intervals, by single year of age, sex and local authority?
- Discussion
- Annex A – Methods for measuring statistical uncertainty in our mid-year estimates (MYEs)
- Annex B – List of local authorities’ 2011 and 2016 admin-based population estimates (ABPE) position relative to the 2011 and 2016 mid-year estimates’ uncertainty
- Annex C – List of local authorities with admin-based population estimates above their uncertainty bounds
- Annex D – Methodology for measuring 2011 Census uncertainty at single year of age
1. Introduction
We are committed to maximising our use of administrative data and reducing reliance on the decennial census. In 2019, a third version of admin-based population estimates for England and Wales (ABPE V3.0) was published as research statistics. This report provides insights on ABPE quality by considering measures of statistical uncertainty.
We define statistical uncertainty as the quantification of doubt about an estimate. Research into statistical uncertainty is conducted by Office for National Statistics (ONS) Methodology in collaboration with the University of Southampton Statistical Sciences Research Institute.
The ABPE V3.0 has produced population estimates for 2011 and 2016, building on knowledge gained from previous versions of the methodology, ABPE Version 1 (V1.0) and ABPE Version 2 (V2.0). The explicit design objective for ABPE V3.0 was to avoid population overcount, by introducing an "activity" based metric. The analysis presented in this paper shows that this has not been fully achieved.
The data suggest that in 240 local authorities there is at least one year of age for either males or females where the ABPE overcounts the population. There is overestimation at more ages in Inner London; for example, Newham, Tower Hamlets and Lambeth have overcount in ABPEs for 64, 48 and 39 single years of age, respectively.
For 65% of all ages, ABPE uncertainty intervals entirely contain the mid-year estimate uncertainty intervals, implying that they are both capturing the same "truth", by very different methods. This occurs more often for males (68%) than for females (62%).
ABPE overcount is concentrated among under-ones, children aged 5 to 18 years and pensioners. These ages need further investigation.
There are characteristic patterns of undercount in the ABPE, particularly at student ages. In four local authorities, the ABPEs are at least 15% lower than our uncertainty measures suggest they should be.
The relationship between local authority mid-year estimates (MYEs) and ABPEs has shifted substantially between 2011 and 2016. ABPEs appear to align more closely with the MYEs in 2016 than they did in 2011, with 14% of ABPEs falling within the MYE uncertainty bounds in 2011 and 45% in 2016. Further research should investigate the relationship between the ABPEs and the true population count over time.
The coverage assessment process for ABPEs will be challenging, particularly when time lags in the administrative data mean that people will be counted in the wrong place. We recommend that further design of the ABPEs, and the inclusion rules for each demographic group, should be closely informed by the proposed coverage assessment strategy for that group.
Nôl i'r tabl cynnwys2. Acknowledgements
We would like to acknowledge Professor Peter Smith from the University of Southampton Statistical Sciences Research Institute who has helped us to develop the measures of statistical uncertainty described in this paper. We are also indebted to him for his comments and suggestions in the research and writing of this report.
Nôl i'r tabl cynnwys3. Disclaimer
The admin-based population estimates (ABPEs) are research outputs and not official statistics. They are published as outputs from research into a methodology different to that currently used in the production of population and migration statistics. As we develop our methods, we are also developing the ways we understand and measure uncertainty about them. These outputs should not be used for policy- or decision-making.
Nôl i'r tabl cynnwys4. Design of the admin-based population estimates and their statistical uncertainty
The admin-based population estimates (ABPEs) are produced through linkage of administrative data and the application of a set of rules in the attempt to replicate the usually resident population. The sources used in the ABPE Version 2 (V2.0) were the NHS Patient Register (PR), the Department for Work and Pensions (DWP) Customer Information System (CIS), data from the Higher Education Statistics Agency (HESA) and data from the School Census (SC). Records found on two of these four data sources were included in the population. This led to estimates that were higher than the official estimates, especially for males of working age.
The main design objective of the ABPE Version 3 (V3.0) was to remove records that were erroneously included in the previous method. An ABPE with under-coverage for all age and sex groups would be closer to unadjusted census counts and, when combined with a Population Coverage Survey (PCS), should allow dual-system type estimators to be applied with improved results. The new method thus uses a different approach – utilizing additional data sources and introducing stricter criteria for inclusion in the population.
The data sources used in the new method are:
Pay As You Earn (PAYE) and Tax Credits data
National Benefits Database (NBD) and Housing Benefit (SHBE) data
Child Benefit data
NHS PR and Personal Demographic Service (PDS) data
HESA data
English and Welsh SC data
Births registrations data
The new criteria used for inclusion in the population are:
a sign of activity within the 12 months prior to the reference date of the ABPE, where by activity we mean an individual interacting with an administrative system (for example, when paying tax or changing address)
appearance and activity on a single data source, with data linkage only used to deduplicate records that appear on more than one source
More details about the choice of data sources and the criteria for inclusion in the new ABPE method can be found in the Principles of ABPE V3.0 methodology.
The new methodology has produced population estimates for 2011 and 2016, where the 2011 reference date is 27 March (Census date), and the 2016 reference date is 30 June (Mid-Year Estimates (MYEs) reference date).
The analysis presented in this paper provides an insight on the quality of the ABPE V3.0 using newly developed measures of statistical uncertainty. These will feed into the evaluation of the ABPE V3.0 and will inform the development of the next iteration.
The measures of statistical uncertainty described in this paper were developed as part of a wider Uncertainty Project that we are conducting in collaboration with the University of Southampton Statistical Sciences Research Institute. The project aims at providing users of our population and migration statistics with information about their quality. The project has been successfully applied in the context of mid-year population estimates and has more recently been applied to admin-based population estimates.
Nôl i'r tabl cynnwys5. Comparison of ABPEs with official population estimates time series
Here we compare the admin-based population estimates (ABPEs) for 2011 and 2016 with the published Office for National Statistics (ONS) population estimates for 2011 to 2016, at the local authority (LA) level. The latter include 2011 Census estimates and 2011 to 2016 mid-year population estimates (MYEs), together with the MYEs measures of statistical uncertainty. Details of the methods used to measure uncertainty in the MYEs are available in Methodology for measuring uncertainty in ONS local authority mid-year population estimates: 2012 to 2016 and Guidance on interpreting the statistical measures of uncertainty in ONS local authority mid-year population estimates. They are also summarised in Annex A, for reference.
Statistical uncertainty in local authority MYEs, 2011 to 16
A major statistical concern with the design of the local authority mid-year population estimates (MYEs) is that their quality decreases with time following the census. Statistical uncertainty in local authority MYEs grows each year between 2011 and 2016. Table 1 confirms that in 2011 the mid-year estimate uncertainty intervals were at their narrowest, with 330 local authorities having 95% uncertainty intervals of less than 5% of their mean simulated mid-year estimate values.
Year | Uncertainty interval range (%) | <5% | 5 to less than 10% | 10 to less than 20% | 20 to less than 50% | ≥50% |
---|---|---|---|---|---|---|
2011 | 1.19 to 7.35 | 330 | 18 | 0 | 0 | 0 |
2012 | 1.42 to 21.24 | 318 | 28 | 1 | 1 | 0 |
2013 | 1.56 to 41.30 | 297 | 44 | 6 | 1 | 0 |
2014 | 1.77 to 44.92 | 290 | 48 | 9 | 1 | 0 |
2015 | 1.85 to 45.58 | 278 | 54 | 15 | 1 | 0 |
2016 | 1.93 to 47.40 | 262 | 65 | 19 | 2 | 0 |
Download this table Table 1 : 2011 to 2016 empirical local authority 95% uncertainty interval range, as a percentage of the mean of the simulated composite mid-year estimates
.xls .csvInitially most uncertainty comes from the census, but each year more uncertainty comes from internal and international migration. In 2012, for most local authorities (330 out of 348), the greatest proportion of uncertainty came from the census (see Methodology for measuring uncertainty in ONS local authority mid-year population estimates: 2012 to 2016, Section 6). The influence of the census declines over time. By 2016, census accounted for 50% of uncertainty in 155 local authorities. The influence of international and internal migration becomes more visible. In 2016, international migration accounted for more than 50% of uncertainty in 93 local authorities, while internal migration accounted for over 50% in just 17 local authorities.
Over time, a growing number of local authority mid-year estimates fall outside of their uncertainty bounds (Table 2). By 2016, over a third of local authority mid-year estimates do. This is consistent with our understanding that estimation of the population becomes progressively more difficult as we move away from the census. However, it could possibly be an artefact of the methodology for measuring uncertainty in the internal migration component of the MYEs, where the 2011 Census internal migration transitions are used as a benchmark of the "true" measure of internal migration (see Methodology for measuring uncertainty in ONS local authority mid-year population estimates: 2012 to 2016, Section 5).
Year | Number within | % | Number above | % | Number below | % |
---|---|---|---|---|---|---|
2011 | 348 | 100.0 | ||||
2012 | 347 | 99.7 | 1 | 0.3 | ||
2013 | 316 | 90.8 | 28 | 8.1 | 4 | 1.2 |
2014 | 271 | 77.9 | 66 | 19.0 | 11 | 3.2 |
2015 | 237 | 68.1 | 95 | 27.3 | 16 | 4.6 |
2016 | 218 | 62.6 | 108 | 31.0 | 22 | 6.3 |
Download this table Table 2: Position of local authority mid-year population estimates relative to their uncertainty intervals, 2011 to 2016
.xls .csvWhat does MYE uncertainty tell us about the local area level ABPEs?
In line with the design objective of undercounting the population, the ABPEs tend to fall below the MYE uncertainty intervals. Table 3 shows that in 2011, 290 (83%) of ABPEs fell below the MYE uncertainty interval. However, nine (2.6%) are above it. The MYE uncertainty bounds are designed to capture 95% of the simulated MYEs. Thus 2.5% of simulated MYEs fall on either side of the uncertainty bounds. Finding 2.6% of ABPEs above the MYE uncertainty bounds would be a welcome finding, except that ABPE V3.0 is designed to deliberately undercount the population.
2011 ABPE | 2016 ABPE | ||||
---|---|---|---|---|---|
Above MYE UI | Inside MYE UI | Below MYE UI | Total | ||
Above MYE UI | 3 | 6 | 0 | 9 | Frequency |
0.9 | 1.7 | 0.0 | 2.6 | Percent | |
Inside MYE UI | 12 | 30 | 7 | 49 | Frequency |
3.5 | 8.6 | 2.0 | 14.1 | Percent | |
Below MYE UI | 17 | 119 | 154 | 290 | Frequency |
4.9 | 34.2 | 44.3 | 83.3 | Percent | |
Total | 32 | 155 | 161 | 348 | Frequency |
9.2 | 44.5 | 46.3 | 100.0 | Percent |
Download this table Table 3: Frequency and percent of local authorities where the ABPEs for 2011 and 2016 fall in or outside of the same year’s mid-year estimate uncertainty interval
.xls .csvTable 3 also shows that the ABPEs appear to align more closely with the MYEs in 2016 than they did in 2011. In 2011, only 14% of ABPEs fell within the MYE uncertainty bounds. By 2016 this rises to 45%. We know that MYEs have increasing bias through the decade after census. If we assume that the accuracy of the ABPE is stable over time, this would imply that MYEs are increasingly underestimating the population. Further research should investigate the relationship between the ABPEs and the true population count through time. This reinforces an important message in Developing our approach for producing admin-based population estimates, subnational analysis: 2011.
A listing of the local authorities in each cell of Table 3 is given in Annex B. Two illustrative examples are presented in Figures 1a and 1b.
Figure 1a: ABPE within MYE uncertainty bounds in 2011 and 2016, Lincoln
Source: Office for National Statistics
Notes:
- Figures standardised to 2011 Census.
Download this chart Figure 1a: ABPE within MYE uncertainty bounds in 2011 and 2016, Lincoln
Image .csv .xls
Figure 1b: ABPE below MYE uncertainty bounds in 2011 and above in 2016, Merton
Source: Office for National Statistics
Notes:
- Figures standardised to 2011 Census.
Download this chart Figure 1b: ABPE below MYE uncertainty bounds in 2011 and above in 2016, Merton
Image .csv .xls6. What can we learn from statistical uncertainty in the ABPEs?
We have produced indicative measures of statistical uncertainty for the 2011 admin-based population estimates (ABPEs). These are interim measures; ultimately confidence intervals will be generated as part of the ABPEs coverage assessment process.1
Methodology for measuring statistical uncertainty in the ABPEs
Our approach relies on two simplifying assumptions. First, that we can use the variability between the ABPE and census estimates within groups of ”similar” local authorities as a proxy for variability of the ABPEs within those local authorities. The grouping of “similar” local authorities is achieved with reference to their patterns of comparability between the ABPEs and census by sex and single year of age. Second, that we can use 2011 Census estimates to represent the true population. Thus, our method doesn’t consider uncertainty in the 2011 Census estimates.
A full account of our methodology is provided in Indicative uncertainty intervals for the admin-based population estimates: July 2020. It can be summarised by the following process:
- calculate scaling factors comparing ABPE and Census by sex and single year of age for each local authority
- normalise the scaling factors around zero using the logarithmic transformation
cluster local authorities based on similar patterns of logged scaling factors across age, for each sex separately
for each cluster, fit a Generalised Additive Model through the lsf, to obtain the model residuals (error), ri,j,k
for each year of age and sex within a cluster, treat as a “group” and produce standardised residuals (s) by dividing them by their group’s standard deviation
- resample 1,000 standardised residuals (with replacement each time)
- un-standardise the residuals by multiplying by their group’s standard deviation
- add the residuals to the observed lsfs in each group to create 1,000 simulated lsfs
- exponentiate the simulated lsfs and multiply them by the published ABPE
- the uncertainty interval is taken as the 2.5th and 97.5th percentile of the 1,000 simulated population estimates
A small number of local authorities could not be clustered with others as they had distinct and unique scaling factor profiles. In these local authorities, the ABPEs often perform less well than in the others, for example, because the administrative sources don’t include foreign armed forces and their dependents. These “outlier” local authorities were grouped within their own separate clusters (separately for males and females) and, appropriately, have larger uncertainty intervals as a result. The outlier local authorities for males were Isles of Scilly, City of London, Forest Heath, Kensington and Chelsea and Rutland. For females the outliers were Isles of Scilly, City of London, Kensington and Chelsea, Forest Heath and Westminster. For further discussion about areas with large populations of armed forces (for example Forest Heath and Rutland) and the quality of the associated ABPEs see also Developing our approach for producing admin-based population estimates, subnational analysis: 2011.
2011 ABPE uncertainty by single year of age, sex and local authority
If the assumptions we have made in estimating uncertainty are correct, we would expect these intervals on average to capture the true population 95% of the time. Uncertainty interval widths for the ABPE reflect known patterns of statistical uncertainty in particular age-sex groups and are calculated as a percentage of ABPE. The intervals are on average wider at student ages and up to age 40 years, just before the retirement age and in the oldest ages. Figure 2 shows the average relative interval widths by age for males. The patterns are nearly identical for females.
Figure 2: Average ABPE uncertainty relative interval widths by age, males
Source: Office for National Statistics
Download this chart Figure 2: Average ABPE uncertainty relative interval widths by age, males
Image .csv .xlsIn this section we report on the position of the 2011 ABPE relative to their uncertainty intervals.
Local authorities where the ABPEs sit entirely within their uncertainty bounds are not excessively biased at any point in the age distribution. For example, Figure 3 for Newport males. Few local authorities have ABPEs which sit within their uncertainty bounds for all ages; six for males (Kensington and Chelsea, Leeds, Newport, Rutland, Sunderland, Wirral) and two for females (Kensington and Chelsea, Westminster). In Westminster (females) and both males and females in Kensington and Chelsea, uncertainty intervals are especially wide (see Figure 4 for Kensington and Chelsea females).
Figure 3: ABPEs and their uncertainty bounds for Newport, males
Source: Office for National Statistics
Download this chart Figure 3: ABPEs and their uncertainty bounds for Newport, males
Image .csv .xls
Figure 4: ABPEs and their uncertainty bounds for Kensington and Chelsea, females
Source: Office for National Statistics
Download this chart Figure 4: ABPEs and their uncertainty bounds for Kensington and Chelsea, females
Image .csv .xlsLocal authorities where the ABPEs are above their uncertainty bounds may be over-estimating the population at those ages. This typically occurs between ages 6 and 24 years, or around the pension age, and is equally common for males (193 local authorities) and females (191). This happens in five scenarios.
Scenario one
Most commonly, primary age children of both sexes (up to 11) may be being over-estimated. This affects most London boroughs, many urban boroughs in the North, a substantial number of urban and suburban local authorities and a few rural local authorities (see Annex C). 138 local authorities have at least one instance of overcount for boys up to 11 years, compared with 121 for girls. Females in the London Borough of Ealing is an example of potential primary age overcount (Figure 5).
Figure 5: ABPEs and their uncertainty bounds for the London Borough of Ealing, females
Source: Office for National Statistics
Download this chart Figure 5: ABPEs and their uncertainty bounds for the London Borough of Ealing, females
Image .csv .xlsScenario two
In some local authorities ABPEs are above their uncertainty bounds in adolescent years (ages 11 to 17 years). This occurs for girls in 57 local authorities and for boys in 45, again listed in Annex C. Males in the London Borough of Wandsworth are an example (Figure 6).
Figure 6: ABPEs and their uncertainty bounds for Wandsworth, males
Source: Office for National Statistics
Download this chart Figure 6: ABPEs and their uncertainty bounds for Wandsworth, males
Image .csv .xlsScenario three
Some local authorities (listed in Annex C) have ABPEs above the uncertainty intervals at ages 21 to 27 years (39 for females, 10 for males). These tend to have large student populations aged 18 to 21 years, and much smaller populations above undergraduate age. In these areas the ABPE may overcount students whose registration has remained after they have moved out and are no longer students there.
Scenario four
Three local authorities have ABPEs above the uncertainty interval at some other ages. City of London (28 to 37 years), Isles of Scilly (16 to 18 years, 28 to 30 years) and Boston (2 to 17 years, 22 to 38 years). Boston has a very high number of seasonal workers from Eastern Europe (Figure 7).
Figure 7: ABPEs and their uncertainty bounds for Boston, males
Source: Office for National Statistics
Download this chart Figure 7: ABPEs and their uncertainty bounds for Boston, males
Image .csv .xlsScenario five
There are 162 local authorities where there is at least one instance of potential ABPE overcount for age 55 years or older; 108 for females and 116 for males. Table 4 shows local authorities with the highest frequencies.
Local authority | Number of single year of age estimates that are above the upper ABPE uncertainty bound | Average percentage above the upper bound of the uncertainty interval (as a % of the upper bound) |
---|---|---|
Newham | 38 | 2.56 |
Tower Hamlets | 32 | 2.66 |
Lambeth | 28 | 2.55 |
Haringey | 22 | 2.31 |
Hackney | 20 | 2.60 |
Crawley | 14 | 0.88 |
Croydon | 13 | 1.52 |
Lewisham | 12 | 1.40 |
Brent | 11 | 3.68 |
Corby | 11 | 2.62 |
Slough | 11 | 2.61 |
Watford | 11 | 1.60 |
Southwark | 10 | 2.03 |
Barking and Dagenham | 10 | 1.39 |
Reading | 10 | 1.19 |
Download this table Table 4: Local authorities with the highest frequencies of ABPEs by single year of age above their upper uncertainty bound, at age 55 years and over
.xls .csvWhere ABPEs are above their uncertainty interval:
occurs more frequently in urban local authorities, particularly London
occurs most frequently at primary school age, student age (especially females) and post-retirement age
for both males and females there is an increase in the number of local authorities with overcount from retirement age onwards; for females there is an additional increase in cases at 90 years old and above
does not consistently increase by age at high ages
Most local authorities where the ABPEs are below their uncertainty bounds are meeting ABPE design objectives.There are just 11 local authorities where ABPEs do not fall below the uncertainty bounds at any age for males, and eight for females.
ABPEs below the 95% uncertainty interval are concentrated between the ages of 18 to 26 years and 40 to 60 years and are more common and pronounced for males. Table 5 lists local authorities with the highest frequency of undercount, alongside the average percentage by which the ABPE is below the lower bound of the uncertainty interval. Males have more potential for undercount than for females, previously attributed in our 2019 publication to shortfalls in coverage of men in the contributing administrative sources. In some areas this may reflect the presence of Foreign Armed Forces or prisons (see also Developing our approach for producing admin-based population estimates, subnational analysis: 2011). Figures 8 and 9 show Camden females and Tunbridge Wells males.
Local authority | Number of single year of age estimates that are below the lower ABPE uncertainty bound | Average percentage below the lower bound of the uncertainty interval (as a % of the lower bound) |
---|---|---|
Females | ||
Camden | 54 | 5.80 |
Tunbridge Wells | 51 | 4.49 |
Elmbridge | 39 | 6.09 |
St Edmundsbury | 39 | 3.37 |
Shropshire | 39 | 3.35 |
Rutland | 38 | 6.45 |
Gwynedd | 38 | 4.58 |
Mole Valley | 37 | 4.85 |
Tandridge | 37 | 3.90 |
Richmondshire | 36 | 4.62 |
East Cambridgeshire | 36 | 4.31 |
Mid Suffolk | 36 | 3.49 |
South Norfolk | 36 | 3.12 |
Uttlesford | 35 | 5.75 |
Wandsworth | 34 | 4.18 |
Males | ||
Westminster | 62 | 6.73 |
Tunbridge Wells | 51 | 8.99 |
Mid Suffolk | 48 | 5.45 |
North Dorset | 47 | 8.64 |
St Edmundsbury | 47 | 8.46 |
Gwynedd | 46 | 5.03 |
Shropshire | 45 | 9.24 |
Harborough | 45 | 6.84 |
Camden | 45 | 5.52 |
Richmond upon Thames | 44 | 7.98 |
Tandridge | 44 | 7.49 |
Wealden | 44 | 6.67 |
Reigate and Banstead | 44 | 5.97 |
Derbyshire Dales | 43 | 7.26 |
Harrogate | 43 | 5.96 |
Download this table Table 5: Local authorities where admin-based estimates are most frequently below their uncertainty bounds
.xls .csv
Figure 8: ABPEs and their uncertainty bounds for Camden, females
Source: Office for National Statistics
Download this chart Figure 8: ABPEs and their uncertainty bounds for Camden, females
Image .csv .xls
Figure 9: ABPEs and their uncertainty bounds for Tunbridge Wells, males
Source: Office for National Statistics
Download this chart Figure 9: ABPEs and their uncertainty bounds for Tunbridge Wells, males
Image .csv .xlsLocal authorities with differences between the ABPEs and the lower uncertainty bound at ages 18 to 26 years fall into three types.
Those with large student populations
The ABPE spike in the number of 18- to 21-year-olds present is lower than suggested by the 2011 Census and uncertainty bounds. Differences are typically greater for males. Males in Newcastle under Lyme (Figure 10) and females in Bristol (Figure 11) are examples.
Figure 10: 2011 admin-based population estimate uncertainty by age and sex for males in Newcastle under Lyme
Source: Office for National Statistics
Download this chart Figure 10: 2011 admin-based population estimate uncertainty by age and sex for males in Newcastle under Lyme
Image .csv .xls
Figure 11: 2011 admin-based population estimate uncertainty by age and sex for females in Bristol
Source: Office for National Statistics
Download this chart Figure 11: 2011 admin-based population estimate uncertainty by age and sex for females in Bristol
Image .csv .xlsThose with high student out-migration
Most local authorities (244) show a distinct drop in 18- to 21-year-olds, suggesting moves for higher education, or work. In some the decrease is much higher in the ABPEs than in the 2011 Census and implied by the uncertainty bounds. For males, 126 local authorities have ABPEs that are on average lower than the lower uncertainty bound. In 53 (listed in Table 6), the ABPE is more than 5% lower. In four, it is more than 15% lower (listed in Table 6). These patterns typically occur in rural areas and appear to be concentrated around wealthier rural or suburban areas (see Shropshire, Figure 12).
Distance from lower uncertainty bounds | Local authorities |
---|---|
More than 5%, females | None |
More than 5%, males | Aylesbury Vale, Bexley, Blaby, Braintree, Breckland, Bridgend, Bromsgrove, Craven, Dartford, Derbyshire Dales, Dover, East Devon, East Hertfordshire, Eastleigh, Epping Forest, Flintshire, Hambleton, Harborough, Harrogate, Hart, Herefordshire, High Peak, Huntingdonshire, Lewes, Mid Suffolk, Mole Valley, Monmouthshire, North Devon, North Dorset, North Hertfordshire, Pembrokeshire, Powys, Purbeck, Reigate and Banstead, Rushmoor, Sevenoaks, Shepway, Shropshire, South Oxfordshire, South Staffordshire, St Edmundsbury, Staffordshire Moorlands, Tandridge, The Vale of Glamorgan, Three Rivers, Tonbridge and Malling, Tunbridge Wells, Uttlesford, West Devon, West Oxfordshire, Wiltshire |
More than 15%, females | None |
More than 15%, males (shown as a percentage of the lower bound value) | Hambleton (15.90), North Dorset (23.89), Shropshire (22.14), South Staffordshire (17.95) |
Download this table Table 6: Local authority distances from the lower ABPE uncertainty bounds
.xls .csv
Figure 12: 2011 admin-based population estimate uncertainty by age and sex for males in Shropshire
Source: Office for National Statistics
Download this chart Figure 12: 2011 admin-based population estimate uncertainty by age and sex for males in Shropshire
Image .csv .xlsOther specific contexts
In a small number of local authorities, the ABPE underestimates young men in rural areas with an army base. In the London boroughs the ABPE appears to underestimate the number of 18- to 30-year olds (listed in Table 7).
Sex | Local authorities |
---|---|
Only Males | Barnet, Bromley, Camden, Greenwich, Hammersmith and Fulham, Haringey, Harrow, Islington, Kingston upon Thames, Lewisham, Merton, Newham, Redbridge, Richmond upon Thames, Waltham Forest, Westminster |
Only Females | None |
Both | Bexley, Croydon, Enfield, Hackney, Havering, Lambeth, Southwark, Sutton, Wandsworth |
Download this table Table 7: Potential undercount in London boroughs at age 18 to 30 years (undercount for at least three consecutive ages)
.xls .csvLocal authorities with substantial differences between the ABPEs and the lower uncertainty bound for 30- to 60-year-olds are mostly in the South, particularly the Home Counties. Demographically, these also fall into two types.
Rural or semi-urban areas
Rural or semi-urban areas with low numbers of 18- to 22-year-olds, together with a large number of 40- to 55-year-olds. ABPEs outside of the uncertainty bounds tend to be concentrated around ages 40 to 60 years. For females, 173 local authorities had ABPEs below the lower uncertainty bound for at least 15 ages in this age range. For males this was 168. There are a few local authorities with a gap between the lower uncertainty interval and the ABPE, but where there are also high numbers of 18- to 22-year-olds. This happens when a local authority encompasses a university town and a large surrounding rural area.
London boroughs
London boroughs where the ABPEs fall below the lower uncertainty bound at ages 30 to 45 years are listed in Table 8. Typically, these areas have large populations aged in their 30s. It is unclear why London suburbs are much more affected by this compared with suburbs of other cities.
Sex | Local authorities |
---|---|
Only Males | Westminster |
Only Females | Barking and Dagenham, Ealing, Hounslow, Islington, Tower Hamlets |
Both | Barnet, Bexley, Bromley, Camden, Croydon, Enfield, Greenwich, Hackney, Hammersmith and Fulham, Haringey, Harrow, Havering, Kingston upon Thames, Lewisham, Merton, Redbridge, Richmond upon Thames, Southwark, Sutton, Waltham Forest, Wandsworth |
Download this table Table 8: Potential undercount in London borough age 30 to 45 years (undercount for at least three consecutive ages)
.xls .csvAfter age 65 years, the number of local authorities with any undercount decreases, from roughly 400 observations of undercount at each age for both sexes leading up to age 65 years, to around 20 for each age after 65 years.
To conclude this section, what if our central assumption that we can use variability between the ABPEs and census within groups of “similar” local authorities as a proxy for variability of the ABPEs within those local authorities, is wrong? We also assume that the census represents the “true” population, with no account taken of uncertainty around the census estimates themselves. We tested our findings by comparing ABPEs against census estimates at single year of age with their associated uncertainty bounds. The methods are shown in Annex D. The results from this analysis reinforce all the findings within this section.
Notes for: What can we learn from statistical uncertainty in the ABPEs?
- We acknowledge that there are minor differences in the 2011 ABPE data for 0-years old that we used for the analysis in Sections 6 to 7 of this paper and those that were used in Developing our approach for producing admin-based population estimates, subnational analysis: 2011 and Measuring and adjusting for coverage patterns in the admin-based population estimates, England and Wales: 2011. This reflects that when we started this work, we had an earlier extract of the data. The differences do not impact the uncertainty measures or any substantive points in the report.
8. Discussion
Admin-based population estimates (ABPE) Version 3 (V3.0) had a specific objective to remove the population overcount seen in ABPE Version 2 (V2.0). The analysis in this paper shows that this objective has not been fully met. There were 38 local authorities with ABPEs above the mid-year population estimates (MYEs) and their uncertainty bounds in either 2011 or 2016 or in both years. Developing methods to avoid ABPE overcount requires further research (see Measuring and adjusting for coverage patterns in the admin-based population estimates, England and Wales: 2011).
At local authority level the relationship between the ABPEs and MYEs has shifted over time. While 5% of ABPEs (19 of 348) were higher than the MYEs in 2011, by 2016 this increases to 19% (67 of 348). We show that the percentage of local authority ABPEs falling below the MYE lower uncertainty bound fell from 83% to 46% between 2011 and 2016. We know that the inter-censal estimates suffer increasing bias over time, largely because of reliance on the International Passenger Survey for measuring international migration (see also Section 5). In addition, internal migration may not be accurately captured. The closer alignment of ABPEs and MYEs in 2016 could be a product of increasing bias in the MYEs. However, we cannot rely on the untested assumption that the relationship between the true population and the administrative sources is constant over time. This requires further research. Time series analysis of the ABPEs and of the administrative sources at aggregate level, prior to any record linkage, would help to signal any change in quality if trends in one source are not visible in others (see also Developing our approach for producing admin-based population estimates, subnational analysis: 2011).
More granular analysis by single year of age and sex reveals the minimum degree of potential overcount in the ABPEs. Measured across local authorities, on average 15 single year of age estimates for females and 14 for males were above the upper bound of the MYE uncertainty interval. Comparing the ABPE and MYE uncertainty intervals, these were found not to overlap for 3.4% of single year of age estimates. This implies that over 96% of ABPEs may capture the same “true” population estimate at single year of age and sex. This does not necessarily imply that they capture the same individuals, for example, see Measuring and adjusting for coverage patterns in the admin-based population estimates, England and Wales: 2011 for compensating over- and under-count errors when linked to the census.
ABPE overcount is concentrated among the under-ones, children aged 5 to 18 years and pensioners. Overcount for each of these age groups requires further investigation (for pensioners it is discussed in more detail in Developing our approach for producing admin-based population estimates, subnational analysis: 2011). Are the ABPEs including people who are not usual residents? Or are some records being double-counted? Or are both happening? The overcount raises questions about whether the “activities” detected in administrative data really signal usual residence, and whether inclusion rules around co-resident inactive records are maybe too relaxed? How much linkage error is attributable to poor date of birth capture in the respective sources? These findings and the questions that they raise are consistent with those in Measuring and adjusting for coverage patterns in the admin-based population estimates, England and Wales: 2011.
Potential undercount in the ABPEs is highest at student ages (18 to 22 years), particularly for males, then falls, then increases again through working ages. This is notoriously a challenging group to capture in population estimates. The pattern is uneven across local authorities with universities. Do we have all the administrative sources that we need for this age group? How far can this undercount be explained by the rules excluding co-resident inactive adult children? 2011 Census data could inform this. Are the high levels of undercount seen in some local authorities correctable through coverage adjustment, and if so, how wide would the associated confidence intervals be for these ages?
The coverage adjustment challenge is more complex at sub-national level than at the national level. This is true for census-based estimates as well, however, administrative data raise additional challenges. Differential time lags in the administrative sources confound record matching. For matched records, address conflicts place records in the wrong geography. Counting records in the wrong place represents overcount in that location, alongside, potentially, undercount somewhere else. The complexity of adjusting for this in estimation underlines the need for ABPE design and the estimation strategy to be closely interrelated.
Further development of the ABPEs would be supported by use of the Error Framework for Longitudinally Linked Administrative Sources.This would help to ensure that statistical error is optimised for the ABPEs all the way through the production process. For further discussion on this see Developing our approach for producing admin-based population estimates, subnational analysis: 2011.
Likewise, the Error Framework should be rigorously applied to the linked data that form the basis of the ABPEs. Again, this is to ensure that statistical error is optimised for the ABPEs.
Nôl i'r tabl cynnwys9. Annex A – Methods for measuring statistical uncertainty in our mid-year estimates (MYEs)
Mid-year population estimates (MYEs) use a cohort component method. In brief, components of demographic change (natural change (births less deaths), net international migration and net internal migration) are added to the previous year’s aged-on population. As well as adding the net components of change, additional procedures account for special populations (for example, armed forces, school boarders, prisoners). Initial work (see Quality measures for population estimates) identified the census base, international migration and internal migration as having the greatest impact on uncertainty, and our measure of uncertainty is a composite of uncertainty associated with these three components only.
Uncertainty can arise from data sources or from the processes used to derive the MYEs. We use observed data and recreate the MYEs’ derivation processes for the three components 1,000 times to simulate a range of possible values that might occur. Differences in data sources and procedures for each component imply different methods to generate the simulated distributions (see Methodology for measuring uncertainty in ONS local authority mid-year population estimates: 2012 to 2016 for details).
The simulated distributions are combined with the other components of change (assumed to have zero error, including births, deaths, asylum seekers, armed forces and prisoners). The uncertainty generation process is summarised in Figure 17. As with the MYEs themselves, the simulated estimates are rolled forward annually through the ten-year inter-censal period. Thus, we include both uncertainty carried forward from previous years (including from the census estimates) and new uncertainty for the current year.
Empirical uncertainty intervals for each local authority are created by ranking the 1,000 simulated values and taking the 26th and 975th values as the lower and upper bounds respectively. As the observed MYE generally differs from the central or median of the simulations, this confidence interval is not centered about the MYE and in some extreme cases the MYE is outside the uncertainty bounds.
Further details of the methods used to measure uncertainty in the MYEs are available in Methodology for measuring uncertainty in ONS local authority mid-year population estimates: 2012 to 2016 and Guidance on interpreting the statistical measures of uncertainty in ONS local authority mid-year population estimates.
Figure 17: The mid-year estimate cohort component method and statistical uncertainty
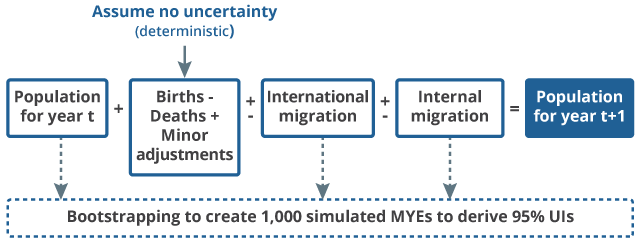
Source: Office for National Statistics
Download this image Figure 17: The mid-year estimate cohort component method and statistical uncertainty
.png (18.2 kB)12. Annex D – Methodology for measuring 2011 Census uncertainty at single year of age
Standard deviations for census estimates at single year of age are not available. We therefore assume that the coefficient of variation is the same for the single years of age as for the corresponding five-year age group. This allows us to estimate the standard deviation by single year of age1:
Therefore
The 2011 Census estimates by single year of age, sex and local authority, and estimated standard deviation by single year of age, sex and local authority are used to specify the distribution (assumed to be normal) of uncertainty around the census component. Parametric bootstrapping from this normal distribution creates 1,000 simulations for the census component for each local authority by single year of age and sex.
Notes for: Annex D – Methodology for measuring 2011 Census uncertainty at single year of age
- This approach is based on an analysis of five-year (published) and single-year (simulated) standard deviations from the 2011 Census, documented in minutes of the meeting between the University of Southampton Statistical Sciences Research Institute and Office for National Statistics on 25 July 2018.