Cynnwys
- Abstract
- Introduction
- What are financial derivatives?
- European Market Infrastructure Regulation trade repository data
- Considerations for applying EMIR in the national accounts
- Alternative data sources for derivatives
- Proposal for the joint application of derivatives data sources
- Author
- References
- Annex A: “Basic” and “enhanced” data cleaning procedures
- Annex B: Illustration of EMIR coverage issue
- Annex C: Raw EMIR data quality concerns
- Annex D: Alternative data sources for derivatives and their comparison to EMIR
1. Abstract
The European Market Infrastructure Regulation (EMIR) dataset is a regulatory dataset on derivatives trades. We have undertaken a comprehensive review into the value of it to compiling derivatives statistics in the UK National Accounts.
Through a combination of data analysis and speaking to industry experts, we have identified the merits and weaknesses of the data in relation to its potential application in the financial accounts.
While the data provide highly granular information about the derivative contracts held by UK entities, we have found that the scope and coverage of contracts reported under EMIR is different from what the national accounts require. There are some overlaps between the two measurement concepts but currently there is no way to reconcile them fully. This represents a major limiting factor in considering the data’s application in the national accounts.
We have also, consistent with the literature, found issues with the quality of the raw data especially in the trade ID and valuation fields. However, we are able to significantly improve the quality of the final data by using a data cleaning methodology that utilises the high-quality central counterparties’ reports in the EMIR two-sided reporting regime.
Overall, as a result of coverage differences we are not confident in the data’s suitability as a primary source of information for compiling the UK’s sectoral and national derivatives balance sheet.
Other data sources on derivatives exist and could be used to compile derivative statistics. They typically cover specific subsectors of the economy and are of much smaller scale than EMIR. We have reviewed and compared their properties with the EMIR data.
Based on our findings, we identify that the following approach can combine the strengths of the different datasets for the future compilation of derivative statistics in the national accounts: where alternative datasets to EMIR exist at the sectoral level and are superior in terms of data quality and consistency with national accounts definitions, they should be used as the primary sources; and where granular breakdowns are not available from the primary source, the EMIR data can be used as a secondary data source to inform the splits.
We note that the EMIR data are increasingly used by central banks and authorities in Europe as a tool in financial stability policy-setting; we are explicit that the findings in this article do not represent the views of the Bank of England and are in no way passing direct or indirect judgement on the data’s usefulness for other policy purposes.
Nôl i'r tabl cynnwys2. Introduction
The Office for National Statistics (ONS) is undertaking the enhanced financial accounts (EFA) initiative in partnership with the Bank of England and Financial Conduct Authority to improve the quality, coverage and granularity of financial statistics, and to provide from-whom-to-whom information on the holdings of financial assets and liabilities.
One of the aims of the EFA initiative is to study the feasibility of using non-survey data to populate the financial account. Compared with traditional surveys, administrative, regulatory and commercial data could potentially be obtained in a timelier manner and could contain information with higher granularity.
The European Market Infrastructure Regulation (EMIR) data is a regulatory dataset on financial derivatives that has the potential to replace existing surveys. This article is a follow-up to a previous publication in June 2017 introducing the EMIR data. We report on the latest research findings on the potential application of the EMIR data and other data sources in compiling derivatives statistics in the national accounts.
We are explicit that views expressed here in this article are the ONS’s conclusions and do not represent the Bank of England’s or the Financial Conduct Authority’s views in any ways.
Nôl i'r tabl cynnwys3. What are financial derivatives?
The European System of Accounts (ESA 2010) defines derivatives as financial instruments based on, or derived from, a different underlying instrument. The underlying instrument is usually another financial asset (for example, foreign exchange, interest rate, equities, credit, and others) but may also be a commodity or an index. For this reason, financial derivatives are referred to as secondary instruments. They may also be referred to as hedging instruments since the hedging or offsetting of risk are frequently a motivation for their creation.
Only those secondary instruments that have a market value, because they are tradable or can be offset on the market, are financial assets in the system of accounts and are classified as derivatives. The contracts can be standardised and traded on exchanges (so called “exchange-traded” or ETD derivatives), or they can be bespoke arrangements traded directly between two counterparties (so called “over-the-counter” or OTC contracts).
According to ESA 2010, examples of financial derivatives include:
options
forwards
swaps
forward rate agreements
credit derivatives
credit default swaps
Financial instruments not included in financial derivatives are:
underlying instrument, structured debt securities (combination of debt securities and financial derivatives) where these are inseparable, and the financial derivative prospective return is small with respect to the principal amount
repayable margin payments, which are classified as other deposits or loans
non-negotiable secondary instruments that cannot be offset on the market
gold swaps and securities repos, which are classified as other deposits or loans
commissions to brokers or intermediaries, which are classed as payments for services
The valuation of derivative contracts is typically more complex than other financial instruments, and depending on the contract type, the basis of valuation can be different. For example, an option has value at inception equal to the premium and is always an asset to the buyer and a liability to the seller. The value can drop to zero over the course of the contract if the option becomes unprofitable to execute.
On the other hand, a forward-type contract has zero value at inception, and over the life of the contract it can switch between an asset and a liability for both counterparties, depending on the spot price and the terms of the contract.
The role that derivatives played in leading to the 2008 financial crisis is well documented in the literature, for example, see House of Commons Treasury Committee report (2009), OECD (2013), Li and Marinc (2014).
Nôl i'r tabl cynnwys4. European Market Infrastructure Regulation trade repository data
Policy background
In the aftermath of the 2008 financial crisis, authorities worldwide identified a number of weaknesses in the functioning of derivative markets, such as the lack of recording and central clearing of over-the-counter (OTC) contracts, which make up the majority of trades. In response, at the Pittsburgh Summit in 2009 the leaders of the G20 group of countries committed to improve the over-the-counter derivatives market by reducing systemic risk and increasing transparency.
In 2012, the European Union introduced the European Market Infrastructure Regulation (EMIR) as an implementation of the 2009 G20 commitment. The two main elements of EMIR are the clearing obligation, which requires that specific OTC contracts are cleared through central clearing parties (see this introduction (PDF, 214KB) by the Bank of England), and the reporting obligation, which requires that all EU entities (except natural persons, central banks and other government or EU bodies charged with managing public debt) entering into any derivatives contracts must submit reports of every trade to a trade repository.
By design, the reporting obligation requires each inter-EU trade to be entered twice as separate entries by both counterparties. This unique feature of two-sided reporting can lead to double-counting during aggregation of the data, which we discuss in greater detail. The reporting obligation of EMIR gives rise to a rich transactional level dataset that is the subject of this article.
Trade repository data
The EMIR trade repository data contain two types of reports:
activity reports, which contain information on financial flows, for example, new derivative trades, modifications, and valuation and cancellation updates
state reports, which contain information on financial stocks, that is, all end-of-day outstanding transactions between individual counterparties
In this article we focus on understanding the properties of the state reports and how they could be used to generate derivative balance sheet estimates.
The state reports are generated each day and are available for the Bank of England and Financial Conduct Authority to access with a one-day lag. The reports contain over 100 fields about the counterparties and the contract for each trade, including the following:
name and Legal Entity Identifier (LEI) of the counterparties
domicile country of the entity
details of the transaction, including:
- Unique Trade Identifier
- information on asset class (foreign exchange, interest rates, and so on) and product type (forward, future, swap, option, and so on) either entered directly or using Classification of Financial Instrument (CFI) code
- date information on execution, valuation, maturity, and so on
- currency in which the contract is denominated
- relevant price, for example, forward exchange rate for forwards
- for options, information also includes the type of option (call or put) and style (for example, European)
- risk mitigation, including confirmation details and collateral posted
- information about clearing
- purpose of the report (new trade, amendment, cancellation, and so on)
- venue of execution exchange-traded (ETD) or over-the-counter (OTC))
- central clearing counterparty involved
Data manipulation
The Office for National Statistics (ONS) currently access the EMIR data through seconding staff into the Bank of England to work on a secure server.
In this article, we make use of Quarter 1 (Jan to Mar) 2017 state report data from four of the six approved EMIR trade repositories (TRs) shown in Table 1. The exclusion of CME from the analysis is because of a significant quality issue that applies to all its data before July 2018. The exclusion of the last three TR’s data from the analysis is a result of data lacking on the Bank of England server, but this is not expected to have a significant effect on the findings in this article as they are either irrelevant for the analysis of the UK or too new to contain Quarter 1 2017 data.
EMIR trade repositories | Inclusion in this analysis | Number of rows in Q1 2017 state report |
---|---|---|
DTCC Derivatives Repository Plc (DDRL, previously DTCC Derivatives Repository Ltd.) | Yes | 13,475,347 |
Regis-TR S.A. | Yes | 1,432,408 |
UnaVista Limited | Yes | 9,963,964 |
ICE Trade Vault Europe Ltd. (ICE TVEL) | Yes | 460,237 |
CME Trade Repository Ltd. (CME TR) | No | 26,078,616 |
Krajowy Depozyt Papierów Wartosciowych S.A. (KDPW) | No |
Download this table Table 1: The list of EMIR trade repositories
.xls .csvIn order to convert the data into a flow of funds (FoF) balance sheet matrix, we need to apply a data-cleaning process that removes outliers, maps EMIR variables to national accounts definitions, removes duplicated records that result under EMIR two-sided reporting requirements, and so on. After discussions with Bank of England, in this article we develop and run two such processes, calling them “basic” and “enhanced”.
The enhanced approach provides an edge over the basic approach by making an informed judgement about the quality of trade reports submitted by different EMIR reporters’ different trading arrangements. It is expected to produce more robust national accounts estimates, at least under the current EMIR reporting landscape. The technical details of the two procedures can be found in Annex A.
Using the basic procedure, for Quarter 1 2017 there are just over 22 million records of open trade positions with unique trade ID to analyse. Using the enhanced procedure, there are instead 16 million records of open trade positions with unique trade ID.
Flow of funds matrix using EMIR
Table 2 shows the overall FoF matrix for Quarter 1 2017 in gross values for all derivative asset classes and contract types, produced using the basic data cleaning procedure, with the same sector classification as available in the 2019 experimental flow of funds statistics. It is possible to further compile FoF matrixes at more granular levels, such as by asset class, contract type, currency of contracts, collaterisation and venue of execution. Table 3 shows a similar FoF matrix produced using the enhanced data cleaning approach.
£billion | Liability | ||||||||||||
---|---|---|---|---|---|---|---|---|---|---|---|---|---|
Asset | PC | PNFC | MFI | OFI | ICPF | HH | NPISH | CG | LG | RoW | Unknown | NA | Grand Total |
PC | 0.0 | ND | 0.0 | ||||||||||
PNFC | 0.5 | 23.5 | 5.7 | 76.9 | 0.1 | 21.1 | 127.8 | ||||||
MFI | 0.1 | 29.2 | 359.5 | 773.8 | 54.8 | 0.3 | 4520.9 | 93.8 | 100 | 5932.5 | |||
OFI | 17.4 | 796.3 | 263.7 | 3.1 | 0.0 | 2795.5 | 14.5 | 80.6 | 3971.2 | ||||
ICPF | ND | 49.2 | 6.3 | 0.7 | 9.4 | 0.2 | 0 | 65.8 | |||||
HH | 0.0 | ||||||||||||
NPISH | 0.0 | ND | 0.0 | 0.0 | 0.0 | ||||||||
CG | |||||||||||||
LG | |||||||||||||
RoW | ND | 59.9 | 6828.3 | 18580.7 | 5.5 | 3659.9 | 19.5 | 465.7 | 29619.6 | ||||
Unknown | 0.0 | 88.3 | 15.4 | 0.0 | 24.8 | 0.4 | 1.3 | 130.2 | |||||
NA | 4.7 | 136.5 | 10.4 | 0.0 | 21.4 | 0.7 | 0.3 | 174 | |||||
Grand Total | 0.1 | 111.8 | 8281.7 | 19656 | 64.2 | 0.3 | 11108.8 | 129.2 | 669.1 | 40021.1 |
Download this table Table 2: Flow of funds matrix of all derivatives in £ billion (gross), Quarter 1 (Jan to Mar) 2017 EMIR data, basic cleaning procedure
.xls .csv
£billion | Liability | ||||||||||||
---|---|---|---|---|---|---|---|---|---|---|---|---|---|
Asset | PC | PNFC | MFI | OFI | ICPF | HH | NPISH | CG | LG | RoW | Unknown | NA | Grand Total |
PC | 0.0 | ND | 0.0 | ||||||||||
PNFC | 0.5 | 21.0 | 4.7 | 63.7 | 0.1 | 20.0 | 110.0 | ||||||
MFI | 0.1 | 27.2 | 347.7 | 583.6 | 49.6 | 0.3 | 4024.0 | 92.4 | 96.5 | 5221.3 | |||
OFI | 16.0 | 580.5 | 177.4 | 3.3 | ND | 1712.3 | 15.0 | 26.9 | 2531.5 | ||||
ICPF | ND | 42.2 | 6.4 | 0.7 | 9.2 | 0.2 | ND | 58.8 | |||||
HH | |||||||||||||
NPISH | 0.0 | 0.0 | 0.0 | ||||||||||
CG | |||||||||||||
LG | |||||||||||||
RoW | ND | 49.3 | 2123.8 | 1892.2 | 5.5 | 0.0 | 2212.1 | 17.5 | 25.7 | 6326.1 | |||
Unknown | 0.0 | 86.6 | 15.8 | 0.0 | 23.5 | 0.1 | 1.2 | 127.6 | |||||
NA | 3.6 | 134.5 | 10.3 | ND | 13.00 | 0.6 | 0.3 | 162.3 | |||||
Grand Total | 0.1 | 96.5 | 3336.5 | 2690.4 | 59.1 | 0.3 | 8057.9 | 126.2 | 170.6 | 14537.6 |
Download this table Table 3: flow of funds matrix for all derivatives in £bn (gross), Q1 (Jan to Mar) 2017 EMIR data, enhanced cleaning procedure
.xls .csv5. Considerations for applying EMIR in the national accounts
In assessing the value of the European Market Infrastructure Regulation (EMIR) data to the national accounts, we consider the following four criteria that underpin bringing in new financial data sources to the Office for National Statistics (ONS) as part of the enhanced financial accounts initiative:
coverage of the data
granularity of the data
quality of the data
availability of from-whom-to-whom information
Coverage
The reporting obligation of EMIR applies to all EU entities (except natural persons, central banks and other government or EU bodies charged with managing public debt) entering into any derivatives contracts. This means that all listed and unlisted contracts, even highly bespoke ones, undertaken by all non-exempt UK entities shall be reported. The coverage of the dataset, in terms of derivative instruments and UK economic sectors, should therefore be very close to being comprehensive.
In practice, EMIR reporting is implemented at the legal entity level and activities of firms are identified through the Legal Entity Identifier (LEI) numbers without any branch location information1. Unfortunately, this creates an issue in aligning the reported trades to the national accounts definitions of local and overseas activities.
A UK legal entity can have operations around the world under its overseas branches2, but under EMIR reporting obligation all these activities shall have to be reported under the UK entity and therefore be wrongly attributed to the UK local economy.
Conversely, a non-EU legal entity can have activities in the UK that we want to capture as part of the local economy, but unless these trades are undertaken against a European entity they will not be covered by the reporting obligation under EMIR and therefore unobserved in the data.
Exceptions to this are, for example, when the contracts reference as underlying a UK entity or UK sovereign debt , and where the trades are novated and cleared by a UK central counterparty (CCP). However, even in the latter case, correct sectorisation of these trades to the UK economy would not be possible because they would all appear under a foreign entity. An internal analysis comparing EMIR microdata with a Bank of England survey designed for national accounts confirms substantial discrepancies at the institutional level and that the patterns are consistent with our theoretical predictions.
See Annex B for a detailed discussion on EMIR coverage issues for the national accounts. In our view this is the area that represents the biggest obstacle to applying EMIR data in the national accounts.
Granularity
Currently, the European System of Accounts (ESA 2010) has options (AF.711) and forwards (AF.712) as the sub-categories under financial derivatives (AF.71). The EMIR data can go much further and contain highly granular information on the properties of the instruments, including asset class, contract type, currency, venue of execution, collaterisation, and other technical dimensions.
Table 4 demonstrates the level of granularity available in the data (for venue of execution and collaterisation the available information is more granular than presented, for example, Market Identifier Code (MIC) is available for venue of execution and the amount of margin posted is available as part of the suite of information on collaterisation, but we abstract from those lower level details).
Information on asset class and contract type are either recorded directly in the EMIR data using the categories in Table 4, or for more standard instruments using Classification of Financial Instrument (CFI) codes, which are approved by the International Organisation for Standardisation (ISO) and used to define financial instruments as a uniform set of code for all market participants. Where CFI codes are used, a Bank of England mapping between CFI codes and the previous categories of asset class and contract type is used. Clearly, instrument granularity is a particular strength of the EMIR data.
Asset class | Contract type | Currency | Venue of execution | Collaterisation |
---|---|---|---|---|
Interest rates | Contracts for difference | GBP | OTC | Uncollaterised |
Currency | Option | USD | ETD | Partial |
Credit | Future | EUR | (MIC codes) | One-way |
Commodity | Forward | JPY | Full | |
Equity | Forward rate agreement | etc… | (amount of margin posted) | |
Other | Swap | |||
Other |
Download this table Table 4: instrument granularity in the EMIR data
.xls .csvGranularity of counterparty sector is also available on the EMIR data insofar as the individual LEI numbers can be mapped to Standard Industrial Classification (SIC) codes or ESA 2010 sectors using the Inter-Departmental Business Register (IDBR). The mapping is feasible but not perfect. This is discussed further in a later section on the availability of from-whom-to-whom information.
Quality
There are well documented issues with the quality of the raw EMIR data, for example, see Fache-Rousova and others (2016) (PDF, 898KB). Since then, efforts have been made to improve the data but some issues appear to remain and limit the data's usefulness to the national accounts.
Findings from our study, which consists of both data analysis and meeting key market players, show that some of the concerns raised in Fache-Rousova and others (2016) are still unresolved after the recent upgrading of the regulatory technical standard and implementation technical standard. We identify further issues with the raw data that are specific to its application in the national accounts.
Our main areas of concerns are:
missing records
difficulty in removing paired records
treatment of cleared OTC contracts
incorrect data
After discussions with Bank of England, we judge that the enhanced data cleaning procedure we deploy is able to significantly improve the quality of the final data.
For a more detailed discussion of the raw data issues, please see Annex C.
Availability of from-whom-to-whom information
The EMIR reporting template includes fields on the Legal Entity Identifier (LEI) numbers of the reporting counterparty and the counterparty on the other side of the trade. Through these two fields, which are compulsory and well filled out in the data, from-whom-to-whom information can be derived.
The challenge for applying the EMIR data in national accounts is in converting LEI information into sectors of the economy. To do this, we make use of an existing mapping on the Inter-Departmental Business Register (IDBR) in the ONS that links the LEI number of entities to their assigned SIC codes and, subsequently, economic sectors.
It is straightforward to apply the mapping to the EMIR data, but doing so is not without caveats: SIC code and economic sector on the IDBR are actually assigned at the enterprise level, which sits one level up from the legal unit (equivalent to legal entity) level in the IDBR hierarchy. As the activity of an enterprise is determined by the combined activity of all legal entities that are part of it, the SIC code and sectors of enterprises are not always an accurate reflection of their component legal entities’ activities.
Another caveat of using the IDBR mapping is that pension funds and investment funds, which are legal entities and can have LEI numbers, do not appear on the IDBR as they are not companies. To correctly sectorise pension funds and investment funds on the EMIR data, there would have to be a bespoke mapping that identifies the list of UK LEIs that are funds.
Comparison to current experimental estimates
Table 5 shows the Blue Book 2019-consistent experimental FoF on derivatives for Quarter 1 (Jan to Mar) 2017. Currently, the main data sources are the Bank of England's Derivative Quarterly (DQ) Survey, the ONS's Securities Dealers Surveys (within the Financial Services Survey) and the ONS' s Investment Trusts Surveys. They focus very much on the monetary financial institutions (MFI) and financial institutions except monetary financial institutions and insurance corporations and pension funds (OFI) sectors.
£ billion | Liability | ||||||||||||
---|---|---|---|---|---|---|---|---|---|---|---|---|---|
Asset | PC | PNFC | MFI | OFI | ICPF | HH | NPISH | CG | LG | ROW | UNK | Grand Total | |
PC | |||||||||||||
PNFC | -1.5 | 38.9 | 29.5 | 66.9 | |||||||||
MFI | 21.4 | 498.8 | 721.8 | 89.4 | 0.6 | -6.8 | 1501.3 | 2826.4 | |||||
OFI | 28.0 | 710.6 | 97.8 | 34.2 | 0.0 | 0.2 | 738.2 | 1608.9 | |||||
ICPF | 88.0 | 32.7 | 120.8 | ||||||||||
HH | 1.0 | 0.0 | -0.2 | 0.9 | |||||||||
NPISH | 0.2 | 0.2 | |||||||||||
CG | 1.5 | 0.2 | 1.7 | ||||||||||
LG | |||||||||||||
ROW | 1539.0 | 747.1 | 2286.1 | ||||||||||
UNK | -1.1 | -0.1 | 0.1 | 1.1 | |||||||||
Grand Total | 0.0 | 48.3 | 2876.3 | 1629.2 | 123.6 | 0.5 | 0.1 | -5.6 | 2239.5 | 6911.9 |
Download this table Table 5: Flow of funds matrix for derivatives in £ billion (gross), ONS experimental statistics Quarter 1 (Jan to Mar) 2017, BB19-consistent
.xls .csvWe can see that compared with the EMIR estimates presented in Tables 2 and 3, there are some striking differences.
Previously, Table 2 shows that the overall derivatives balance sheet in Quarter 1 2017, estimated by the EMIR data with the basic data cleaning procedure, stands at just over £40 trillion. After removing the rest of the world to rest of the world (RoW-RoW) contracts, which are not of interest to the national accounts, the remaining balance sheet still stands at about £36.5 trillion. This is nearly five times bigger than the current derivatives balance sheet estimate of about £7 trillion.
Using the enhanced cleaning procedure instead, as shown in Table 3, helps to bring down the overall estimate to £14.5 trillion, or £12.3 trillion after removing RoW-RoW contracts. This is still higher than the current estimate by 70% but is nonetheless significantly lower than without the enhanced data cleaning procedure.
If we compare the from-whom-to-whom (FWTW) cells in Tables 3 and 5, we can see more clearly the drivers of the overall discrepancy. The biggest differences between the two tables appear to come from the interaction between the financial sectors and the rest of the world. National accounts (NA) experimental estimates of RoW derivative assets held against UK MFI and OFI sectors for Quarter 1 2017 are £1.5 trillion and £0.7 trillion respectively, while the EMIR data (with the enhanced cleaning procedure) suggest they are £2.1 trillion and £1.9 trillion instead.
Similarly, NA experimental estimates for RoW derivative liability held against UK MFI and OFI sectors for Quarter 1 2017 are £1.5 trillion and £0.7 trillion respectively, while the EMIR estimates (with the enhanced cleaning procedure) are £4.0 trillion and £1.7 trillion instead.
We can offer several explanations as to why these differences arise. Firstly, a major contributor to the discrepancy is likely the coverage issue, which we discussed earlier in Section 5. Theoretically, the effect of the coverage issue on the direction of bias in the EMIR is ambiguous. By comparing Tables 3 and 5, however, it appears there is an upward bias in the EMIR estimates, meaning they are over-capturing UK entities’ trades. This may not be a surprise given the global reach of some British financial institutions.
Secondly, there could be a degree of underestimate to the current NA experimental estimate for the overall balance sheet. They are generated by surveys that focus on the MFI and OFI sectors while in reality, for example, private non-financial corporations (PNFCs) may enter into a commodity contract with another PNFC or RoW. The effect of this, though, is minor since the EMIR estimates for the counterparty cells not covered in the experimental NA FoF estimates are in general small in magnitude. The treatment of cleared over-the-counter (OTC) contracts may also be a contributing factor as the official estimates, unlike EMIR, are under-capturing central counterparty (CCP) activities.
Thirdly, quality issues could play a part in driving the discrepancy. In Annex C we outline in more detail issues such as limited data validation carried out by the trade repositories (TRs) on the valuation field and so subsequently it can be difficult to ascertain if the reporters are reporting valuation in the right currency, or even in the right unit. The enhanced data cleaning procedure helps here by only keeping records that are judged to have a better chance of being correctly submitted (for example, using CCP’s reports of cleared OTC contracts rather than the clearing members’ reports) but some misreporting may still remain.
Finally, it may be that some trades are incorrectly reported but they have not been identified as outliers by our basic algorithm. Application of more sophisticated data science techniques may help to further eliminate implausible records.
We can see signs of relative weakness in the EMIR data by looking at sectoral net positions. In Table 5, which is based on survey data sources, the asset and liability positions of a sector roughly match each other and the net balances are fairly close to zero.
This observation also holds true at the micro level when looking at firm-level returns. This is expected, because derivative contracts are hedging instruments and are often used to minimise risks resulting from other financial instruments or derivative contracts. For example, a firm that is currently making a loss in a contract that is yet to mature may decide to take out another contract in the opposite direction to bring the net position to zero and break even. Such intuition cannot be observed in Tables 2 or 3 based on the EMIR data. We see that there are clear sectoral imbalances, in particular in the MFI and RoW sectors, even after the enhanced data cleaning procedure. We believe that this points towards weakness in the EMIR data compared with survey sources.
The effect of the new EMIR technical standards
So far in the analysis we have been using Quarter 1 2017 EMIR data as a showcase of the properties of the data. At the end of 2017, new EMIR regulatory and implementation technical standards were introduced, providing better field definitions and adding and removing some fields. When we analyse the Quarter 4 (Oct to Dec) 2017 data, the improvement to certain features of the TR data is noticeable. For example, there has been more consistency to the structure of different TRs’ data making it easier to merge them into one database, and the rate at which some of the data fields are filled properly has gone up (such as the use of LEI in the counterparty fields). However, we have not noticed significant improvement in the data in relation to our concerns for their application in the national accounts.
The issues of pairing amongst inter-EU trades and the high balance sheet estimate have both persisted. The theoretical coverage issue has remained the same as well. Therefore, we think the conclusions that can be drawn from the later data are not so different from the Quarter 1 2017 data which we use.
Summary
In this section we discussed the value of the EMIR data to national accounts under the criteria of coverage, granularity, quality and availability of from-whom-to-whom. We also compare the results from the EMIR data to existing FoF estimates that are generated mainly using several surveys that cover the MFI and OFI sectors.
We find significant issues with aligning the EMIR-reported activities to the national accounts concepts of local and foreign activities. After analysing the data and speaking to practitioners and a trade repository, we also have significant doubts about the quality of the raw data, although this is to a large extent offset by an informed data cleaning procedure that takes advantage of the high-quality central clearing counterparties (CCP) reports in the data. On the other hand, the EMIR data do offer a great degree of granularity on instrument breakdown, and from-whom-to-whom information is available at the entity level.
We notice that the EMIR estimates of the sectoral and economy-wide derivatives balance sheet and the net balance can be quite different from the current NA experimental estimates, especially on the interactions between the financial sectors and the rest of the world. The evidence points towards the EMIR estimates being unsuitable for use as a primary data source in the national accounts.
Notes for: Considerations for applying EMIR in the national accounts
One of the trade repositories, DTCC, used to have a proprietary field on branch location but since the end of 2017 has discontinued it at the request of ESMA. Our analysis shows that, where possible, the use of branch location can improve the matching of reported derivative holdings of UK banks between EMIR data and the Bank of England’s Derivative Quarterly Survey.
Note it is not an issue for firms to book trades under overseas subsidiaries though, as they are separate legal entities and have different LEI numbers.
6. Alternative data sources for derivatives
Alternative derivatives data sources to the European Market Infrastructure Regulation (EMIR) data exist, each with unique properties. It is, therefore, important to consider the optimal use of all available data sources in compiling derivatives statistics for the national accounts that are robust, granular and can provide from-whom-to-whom information. This will involve identifying the sectors in which there are overlaps with the EMIR data in coverage and, where there are overlaps, establishing a hierarchy of dataset application.
The alternatives to the EMIR data that we have identified are the Bank of England’s Derivative Quarterly (DQ) Survey, the ONS’s Financial Services Survey (FSS), the ONS’s Trusts Survey, the Solvency II (SII) administrative data on insurance companies, the ONS’s Pension Funds Survey (PFS), and the ONS’s Trusts Surveys. We have also identified the market in financial instruments directive II (MiFID II) administrative data as a potential source but it was quickly apparent that it lacks important information to be suitable for compiling derivative statistics in the national accounts.
The joint coverage of all the alternative datasets over the economy is shown in Figure 1, and can be considered as very good. The gaps in coverage are mostly over the household and general government sectors, which are not expected to be significant market participants in derivatives. In many of the from-whom-to-whom (FWTW) cells that EMIR data cover, especially the ones that involve a financial sector, there are overlaps with one or more alternative datasets.
Figure 1: the coverage of available data sources on derivatives over the economy
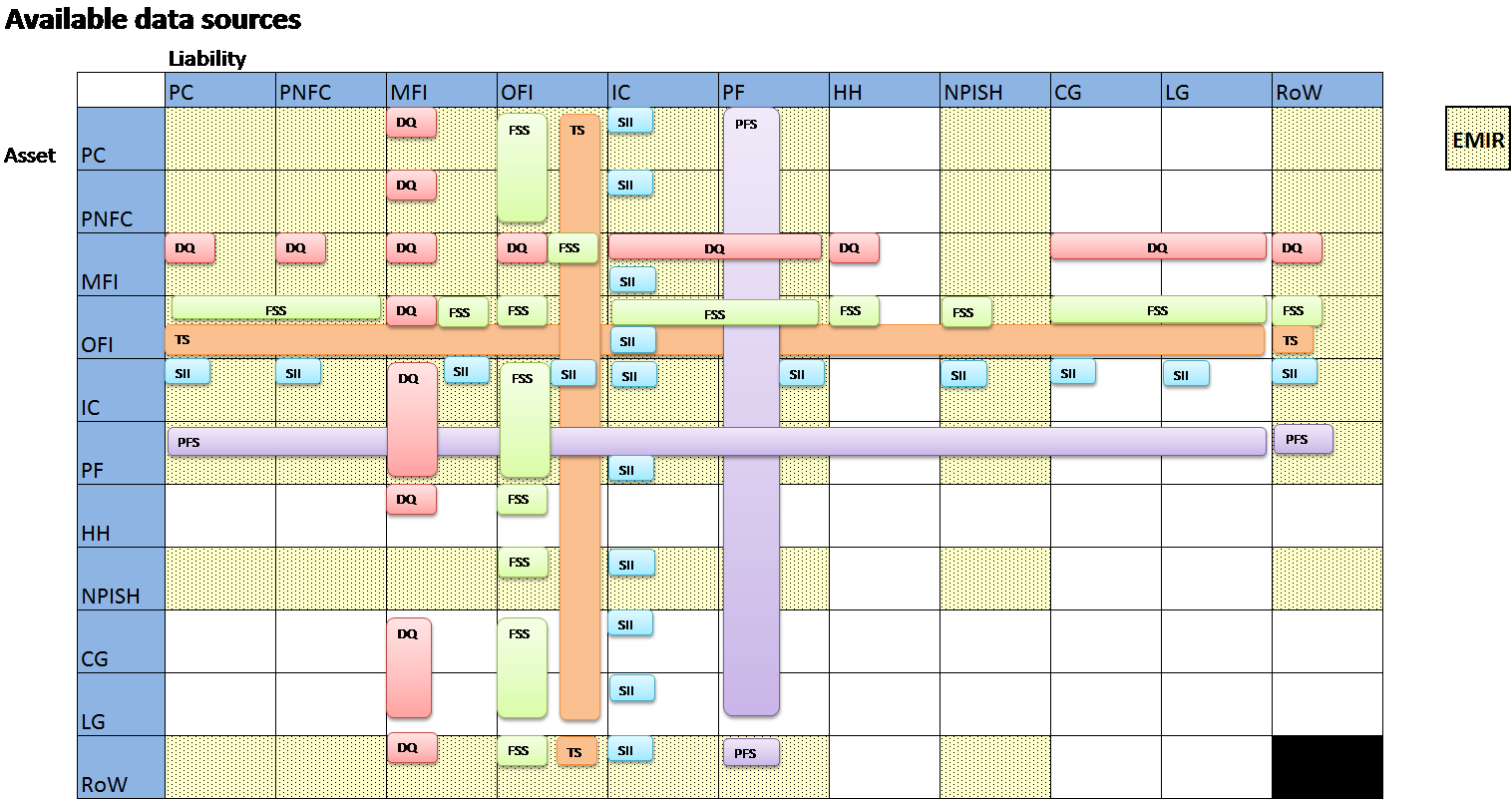
Source: Office for National Statistics and European Market Infrastructure Regulation data
Download this image Figure 1: the coverage of available data sources on derivatives over the economy
.png (110.7 kB)In Annex D we provide a detailed discussion of all the alternative datasets and how they compare with the EMIR data. In general, we find that the alternative datasets are more fitting for use as primary information in the national accounts because of the way the surveys are designed to be consistent with national accounts definitions.
Nôl i'r tabl cynnwys7. Proposal for the joint application of derivatives data sources
We have undertaken a comprehensive review on the value of the European Market Infrastructure Regulation (EMIR) data to compiling derivative statistics in the national accounts. Through a combination of data analysis and speaking to industry experts, we have identified the merits and the weaknesses of the data in relation to their potential application in the national accounts. We have also compared the EMIR data against alternative data sources. We identify that the optimal approach to combining the EMIR data and other data sources in compiling national accounts derivatives flow of funds (FoF) estimates should bear four main elements.
Considerations in combining the data
Element 1: where alternative datasets to EMIR exist at the sectoral level and are superior in terms of data quality and consistency with national accounts definitions, they should be used as the primary sources for estimating the sectoral derivatives balance sheet positions and any granular breakdowns in the from-whom-to-whom matrix
In comparing EMIR estimates with current experimental national accounts (NA) estimates based mostly on form DQ (derivative quarterly), Financial Services (FSS) and Trusts Surveys, we have found discrepancies in certain counterparty sector pairs. We attribute the differences primarily to the misalignment of definitions with NA and secondarily to quality concerns about EMIR fields. Our understanding of the alternative data sources reviewed in this article is that most of them do not suffer from the same coverage issues and are of superior quality to varying degrees in estimating the FoF balance sheets and certain granular breakdowns.
For counterparty cells that are not covered by alternative data sources, the EMIR data could be utilised as the primary data source.
Element 2: where granular breakdowns are not available from the primary source, the EMIR data can be used as a valuable secondary data source to inform the splits
We propose splitting the from-whom-to-whom (FWTW) aggregates over four dimensions, to take advantage of the granular information available in the EMIR data:
asset class (interest rate, foreign exchange, credit, commodities, equity, other products)
contract type (contracts for difference, option, future, forward, forward rate agreement, swap, other types)
currency of contracts (pound sterling, US dollars, euro, other currencies)
venue of execution (over-the-counter, exchange traded)
If there is user demand, it is feasible to split the rest of the world (RoW) sector by countries or regions of the world.
The second element of the optimal approach implies that the utilisation of the EMIR data could vary between FWTW cells in the FoF table, depending on the existing granularity of information in the alternative data sources. We expect the utilisation of the EMIR data to be high for the S.124 to S.127 and S.129 subsectors, as Trusts Surveys, FSS and the Pension Funds Survey (PFS) do not contain detailed instrument and/or counterparty breakdown. On the other hand, we expect the utilisation to be lower for the S.122 and S.128 subsectors, as form DQ already contains quite granular breakdowns (except venue and countries or world region of counterparties) while Solvency II (SII) administrative data are equally rich as the EMIR data across the proposed dimensions of split.
Figure 2: proposed use of data sources in compiling derivatives FWTW estimates
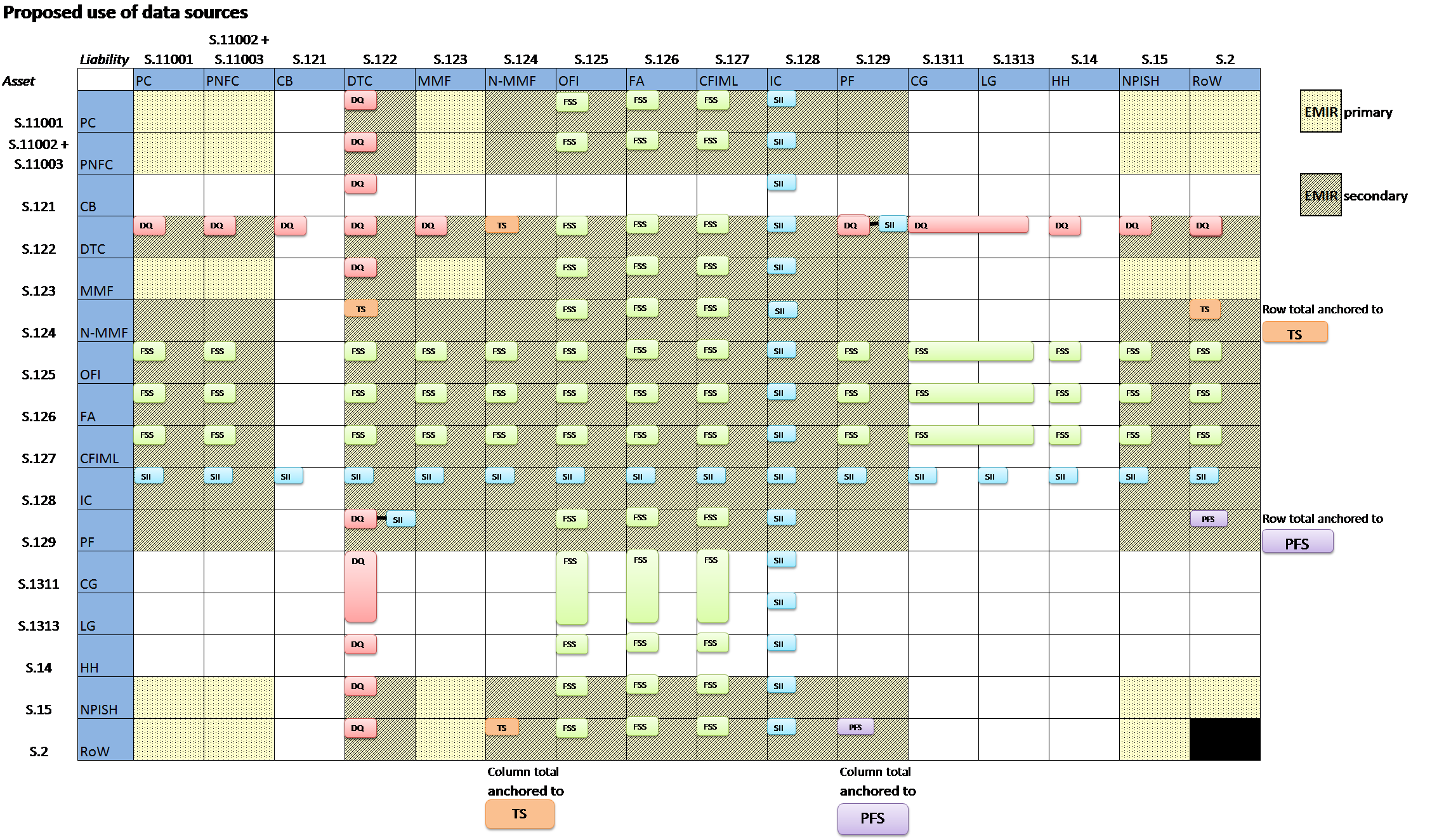
Source: Office for National Statistics and European Market Infrastructure Regulation data
Download this image Figure 2: proposed use of data sources in compiling derivatives FWTW estimates
.png (208.1 kB) .xlsx (23.6 kB)Figure 2 shows a proposal for the combined use of the EMIR data and other data sources in populating the FoF matrixes. It assumes the FSS will be improved from its current version by having more granular counterparty breakdowns (such as splitting from insurance corporations and pension funds).
Note that in Figure 2 there are a number of FWTW cells where we are proposing to combine different data sources in estimating the balance sheet positions.
Firstly, for the S.124 and S.128 subsectors, we are proposing to anchor the column and row totals to the Trusts Surveys and PFS returns, while using DQ, FSS and SII estimates to populate specific FWTW cells where possible. The resulting residuals from deducting DQ, FSS, and SII estimates from the S.124 and S.128 local sectors aggregate will be split over the remaining FWTW cells using weights from the EMIR data. This is so because Trusts Surveys and PFS only ask for aggregates for the local sectors as a whole but lack further granularity.
Secondly, as DQ treats the insurance corporations and pension funds subsectors as one counterparty, we are proposing to estimate S.122-to-S.129 holdings by subtracting the SII S.122-to-S.128 estimates from DQ S.122-to-insurance companies and pension funds (ICPF) estimates. We view this as a superior method to using the EMIR data directly to estimate S.122-to-S.129 balance sheets.
A demonstration of the potential intermediate EMIR output to be generated on and extracted from the BoE server is shown in Table 6. For every end of quarter, we are proposing to aggregate the EMIR data and generate a summary table of EMIR valuations across six dimensions:
asset counterparty sector
liability counterparty sector
asset class
contract type
currency of contract
venue of execution (can be extended to include collaterisation and other fields if there is sufficient user demand)
This approach allows flexible bottom-up aggregation of the splits to the required levels of granularity in complementing alternative data sources.
Date | Asset sector | Liability sector | Class | Type | Currency | Venue | Value (£) |
---|---|---|---|---|---|---|---|
17Q1 | S.122 | S.126 | IR | SW | EUR | ETD | 0.5m |
17Q1 | S.122 | S.126 | FX | FU | USD | ETD | 1.3m |
17Q1 | S.122 | S.2 | CO | OP | GBP | OTC | 0.8m |
17Q1 | S.122 | S.2 | CD | SW | GBP | OTC | 2.5m |
… | … | … | … | … | … | … | … |
Download this table Table 6: A made-up example of EMIR output table to be extracted from the BoE server
.xls .csvElement 3: continue to improve the cleaning and outlier detection procedures for using the EMIR data, with a view to applying data science techniques
Currently, we use a simple rule of thumb to detect valuation outliers in the EMIR data, combining criteria on the absolute size of the valuation and the share that a contract takes up within its specific market. While this is successful at removing some outliers, it does not always work.
For example, if an entity holds many of the same contracts and has mistakenly uplifted all of them by some significant but unknown factor while the valuation of each contract still comes in under the acceptance threshold on size, these trades would pass the current outlier test. In fact, we think at least some of the discrepancies at the micro-level when comparing EMIR and DQ estimates are due to this issue.
The problem is not straightforward to fix, as without exploiting information about other contracts, there is no basis to determine if the valuation of a contract is abnormal. A more sophisticated approach to detecting outliers could be to develop data science methods in order to exploit the high dimensions and sheer volume of the EMIR data in predicting the likely valuation for each trade.
Element 4: continue to follow the policy and user landscapes of EMIR in the next two years to monitor changes to the content and quality of the EMIR data and their value to the national accounts
The sheer volume and the richness of the EMIR data mean that their potential for application in different policy spheres is high. While we currently do not consider the EMIR data suitable as a primary data source in the national accounts, they are very useful tools in financial stability policy-setting and increasingly more and more European central banks and authorities are looking to exploit the data. The ONS should therefore continue to follow EMIR-related policy development and upcoming use cases of the EMIR data by the Bank of England and in other countries in order to reap the benefits of future research on the EMIR data.
Nôl i'r tabl cynnwys9. References
Akritidis, L. (2018) “Experimental financial statistics for insurance using Solvency II regulatory data – enhanced financial accounts (UK flow of funds)”
Evans (2017) “Economic Statistics Transformation Programme: enhanced financial accounts (UK flow of funds) – 2017 matrix update”
Fache-Rousova, L., Osiewicz, M., Kulmala, K.M. (2016) “Reporting of derivatives transactions in Europe – Exploring the potential of EMIR micro data against the challenges of aggregation across six trade repositories”
House of Commons Treasury committee report (2009). “Banking Crisis: regulation and supervision: Responses from the Government and Financial Services Authority to the Committee's Fourteenth Report of Session 2008-09” Treasury Committee Publications
Lai, K. (2017) “Economic Statistics Transformation Programme: enhanced financial accounts (UK flow of funds) progress on financial derivatives data”
Levy, S. (2018) “Pensions in the national accounts, a fuller picture of the UK’s funded and unfunded pension obligations: 2010 to 2015”
Li, S. and Marinc, M. (2014). “The use of financial derivatives and risks of U.S. bank holding companies” International Review of Financial Analysis
Miller, K. (2018) “Economic Statistics Transformation Programme: enhanced financial accounts (UK flow of funds) – shadow banking introductory article”
OECD (2013) “The role of banks, equity markets and institutional investors in long-term financing for growth and development”
Rahman, A. (2015) “Over-the-counter derivatives, central clearing and financial stability”
Nôl i'r tabl cynnwys10. Annex A: “Basic” and “enhanced” data cleaning procedures
The “basic” data-cleaning procedure involves seven steps in converting the European Market Infrastructure Regulation (EMIR) raw data into the flow of funds (FoF) matrix:
Step 1) Basic cleaning and formatting of the raw data within each trade repository (TR)
Step 2) Removing duplicated reports within a TR using trade identifiers. Reporting duplication is expected for inter-EU trades because the reporting obligation of EMIR requires that all non-exempt EU entities report their trades, meaning an inter-EU trade should be reported twice, one time by each counterparty side
Step 3) Aggregating all individual TRs’ data into one dataset and removing inter-TR duplication. This is necessary because counterparties to a trade can choose to report to different trade repositories.
Step 4) Merging TR data with mappings on: a) Legal Entity Identifier (LEI) to Standard Industrial Classification (SIC) codes/European System of Accounts: ESA 2010 sectors of the economy; b) Classification of Financial Instrument (CFI) codes to asset class and contract type, and; c) non-sterling valuation to sterling valuation
Step 5) Assigning asset and liability counterparty for each trade based on the sign of the valuation field
Step 6) Remove outliers according to a simple rule of thumb: trades that are worth over £1 billion or make up over 50% of the market (defined by the counterparty sectors, asset class and contract type) are removed
Step 7) Summing up value of contracts by asset class, contract type and the asset-liability sector pairs The “enhanced” approach involves an extra step 1b (between steps 1 and 2 above) where TR records are first filtered based on the trading arrangement and reporter: a) for over-the-counter (OTC) cleared contracts, trade reports submitted by central clearing counterparties (CCP) are kept while clearing member’s reports are discarded; b) for exchange-traded (ETD) contracts, clearing member’s reports are kept while central counterparty (CCP) reports are discarded; c) for uncleared trades, no filtering is applied.
This modification benefited from discussions with Bank of England and represents the author’s informed judgement about the quality of trade reports submitted by different reporters under different trading arrangements, and the ability to match to CCP public accounts.
Nôl i'r tabl cynnwys11. Annex B: Illustration of EMIR coverage issue
The reporting obligation of European Market Infrastructure Regulation (EMIR) applies to all EU entities (except natural persons, central banks and other government/EU bodies charged with managing public debt) entering into any derivatives contracts. This means that all listed and unlisted contracts, even highly bespoke ones, undertaken by all non-exempt UK entities shall be reported. The coverage of the dataset, in terms of derivative instruments and UK economic sectors, should therefore be very close to being comprehensive.
In practice, EMIR reporting is implemented at the legal entity level and activities of firms are identified through the Legal Entity Identifier (LEI) numbers without any branch location information1. Unfortunately, this creates an issue in aligning the reported trades to the national accounts definitions of local and overseas activities. A UK legal entity can have operations around the world under its overseas branches2, and under EMIR reporting obligation all these activities, whether in EU or not, shall have to be reported.
For example, EMIR captures all derivatives contracts of HSBC plc (a UK legal entity), including those taken up by its overseas branches in all over the world. The national accounts are only concerned with its local activities (as reported by the local reporting units) in the UK and would like to separately classify the activities of its overseas branches as under the rest of the world sector, but since in the EMIR data there is no field to separately identify the location of the contracts, we are unable to assign the trades correctly as local or overseas activities. In this case, to take the LEI-level data on face value will therefore lead to an upward bias in estimating the local activities of UK legal entities.
Conversely, a non-EU legal entity can have activities in the UK that we want to capture as part of the local economy, but unless these trades are undertaken against a European entity they will not be covered by the reporting obligation under EMIR. For example, if the London branch of Citibank N.A. takes out a contract with the London branch of JP Morgan Chase Bank N.A. (both banks are US legal entity), we would want to capture it as an inter-monetary financial institutions trade within the UK, but the trade will not show up in the TR data as it is between two entities not covered by EMIR3. In this case, using EMIR data on face value will lead to an underestimate of UK local activities.
This issue is further exacerbated by the fact that the Bank of England (and by extension the Office for National Statistics (ONS)) only has legal rights to access trade reports that involve a UK counterparty or central counterparty (CCP) or reference as underlying UK entities or UK sovereign debt4. This means that the reports of contracts between non-UK European entities (which can have a strong presence in the UK, such as Deutsche Bank AG, UBS AG, and others) are in general also not accessible to us. Exceptions to this are, for example, when the contracts reference a sterling instrument, and where the trades are novated and cleared by a UK CCP: in the process of novating the contracts, the CCP, which is a UK entity, becomes a counterparty to the trades and hence it would submit reports to a EMIR trade repository.
Figure 4 provides an illustration that shows approximately the misalignment issue of the EMIR data with the national accounts concept of local activities. It is an approximation because it is purely based on the geography in which the activities and the counterparties are based.
We abstract from the subtle points that: 1) contracts referencing UK entities and UK sovereign debt are captured by the EMIR data we can access, regardless of where the counterparties are based; 2) the role that UK CCPs play on capturing over-the-counter (OTC) contracts taken out in the UK by non-UK entities; and 3) UK activities of UK branches of foreign firms are captured to the extent that they involve a UK entity as counterparty although those activities cannot be separated out as mentioned previously.
With those caveats in mind, the largest region in red in Figure 1 represents all the derivative contracts that exist in the global financial system.
The teal region represents the contracts take place within the UK and what the national accounts would consider as within the local economy.
The blue region represents contracts that are reported under EMIR, which are contracts taken out by EU legal entities. They are not the same as contracts that take place within the EU.
Within the blue region of all EMIR reports, the orange region represents EMIR reports that are accessible to the Bank of England, which is the subset of trades that involve at least one UK legal entity. Again, they are not the same as contracts that take place within the UK.
The teal region is what the national accounts try to capture, but instead the EMIR data accessed via the Bank of England covers the orange region, which does not overlap completely with the teal region.
We can immediately see that the EMIR (Bank of England) region is not a good estimate of the national accounts region. They don’t necessarily overlap and the sizes can be quite different as they capture different contracts.
Figure 3: a Venn diagram illustrating the coverage of activities by different definitions
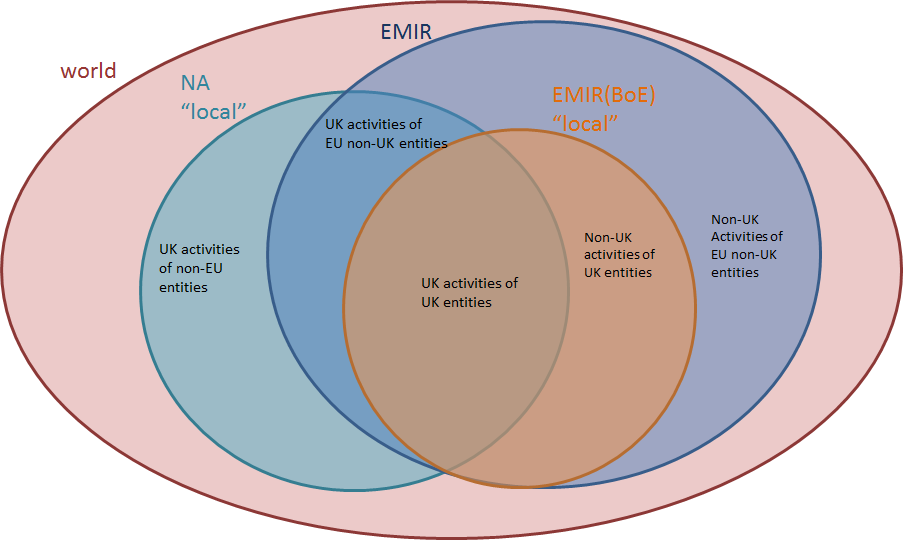
Source: Office for National Statistics
Download this image Figure 3: a Venn diagram illustrating the coverage of activities by different definitions
.png (73.4 kB)The extent to which misalignment of definitions is an issue in practice depends on the degree of the underlap between the teal and orange regions in Figure 4. Theoretically, the more focused the local entities are on the local derivatives market and the more closed the financial system is, the degree of overlap is likely to be high. However, the UK financial system is likely very different from that description: UK banks and other financial institutions have vast overseas presence, while overseas entities and their interactions with each other are a large part of the UK finance industry.
To shed light on the potential extent of the coverage issue, we have carried out an internal analysis of the gross derivative assets and liabilities positions from the EMIR data compared against that from the Bank of England Derivative Quarterly (DQ) Survey for 17 major banks in the UK. As the DQ Survey is designed for national accounting purposes, its estimates can be considered the benchmark in identifying coverage issues in the EMIR data.
We find that substantial differences between the two estimates, ranging from tens of billions GBP to £1 trillion, exist at the institutional level. The coverage issue alone cannot explain all the differences, but the patterns in the results, which we cannot share because of confidentiality agreement, are largely in line with our understanding of the direction of bias that it can cause.
Firstly, the estimates of derivatives assets and liabilities of banks with UK Legal Entity Identifiers (LEIs) are in general higher in the EMIR data than in the DQ Survey. This is likely because of the inclusion of overseas activities of these banks in the EMIR data.
Secondly, the UK subsidiaries of foreign banks have higher balances in the EMIR data. This can be because of the EMIR reporting of contracts that they take on behalf of their foreign parents, which DQ explicitly asks them to exclude. Similarly, EU banks in general also show higher estimates in the EMIR data. This can be because of the EMIR data capturing contracts against UK firms that the overseas headquarters take up, which again are excluded by form DQ. US banks, on the other hand, have lower estimates in the EMIR data. This is expected since their local activities are only covered fully by the DQ Survey not EMIR.
There is no good solution to the coverage issue as within the EMIR data there is currently no flag on the location of activities, and the design of EMIR means local activities of foreign entities in general do not have to be covered,. Even then, since these trades are carried out by an entity with foreign LEI, we would always have to assign them to the RoW sector and are unable to correctly identify which trades are actually local.
Notes for: Annex B: Illustration of EMIR coverage issue
One of the trade repositories, DTCC, used to have a proprietary field on branch location but since the end of 2017 has discontinued it at the request of ESMA. Our analysis shows that, where possible, the use of branch location can improve the matching of reported derivative holdings of UK banks between EMIR data and the Bank of England’s Derivative Quarterly Survey.
Note it is not an issue for firms to book trades under overseas subsidiaries though, as they are separate legal entities and have different LEI numbers.
In reality some still get reported (over-compliance) but they are exceptions.
This was true at the time the analysis was made. Access rights have in part changed in April 2019.
12. Annex C: Raw EMIR data quality concerns
Our main areas of concerns with raw European Market Infrastructure Regulation (EMIR) data are:
missing records
difficulty in removing paired records
treatment of cleared over-the-counter (OTC) contracts
incorrect data
We focus our discussion on the quality of the variables that are critical for compiling the national accounts:
trade identifier
counterparty identifier
product identifier
valuation
Missing records
Since EMIR reporting began in February 2014, there has only been one enforcement action taken by the Financial Conduct Authority (FCA). It was a £34 million fine against Merrill Lynch International for failing to report 68.5 million transactions over a two-year period between February 2014 and February 2016.
Anecdotal evidence from speaking to a major trade repository (TR) suggests that under-compliance is more prevalent than the sole enforcement action would indicate. Firms often have bespoke in-house systems to log derivative transactions for their trading purposes, but these systems rarely speak to the EMIR template directly and so it is costly for firms, particularly ones that are involved in a large number of trades, to develop systems to submit EMIR reports.
The high dimensions of the reporting template, the lack of clarity on the size of fines and the low occurrence of enforcement actions could mean that for some firms it may be cost-efficient to not comply at all. This potentially leads to missing data of non-random nature. Our data cleaning procedure, which filters the raw data based on the credibility of the reporters under different trading arrangements, mitigates this issue to a certain extent.
Difficulty in reconciling records for the same trade using trade ID
The EMIR reporting obligation requires all non-exempt entities in the EU to report their transactions. This means that for an inter-EU trade, the two sides of the contract will both need to submit a report to an EMIR trade repository.
In order to meaningfully aggregate the data to arrive at a sectoral or national level estimate of assets and liabilities, we need to reconcile the information between the duplicated reports and remove one of the pair from the final database. However, as noted by Fache-Rousova and others (2016), pairing rate is low particularly in case of trades reported to different TRs. For the Quarter 1 (Jan to Mar) 2017 data cleaned using the enhanced procedure, the pairing rate for inter-EU trade, defined as the proportion of trades that can be paired by Trade ID, stands at about 40%.
Anecdotal evidence from speaking to a TR suggests one reason for failure to match could be because of situations where the reporting firms submit dummy reports with interim trade IDs to satisfy the timeliness requirement under the reporting obligation, but when they submit the final reports with different and final trade IDs they may not bother to remove the dummy reports.
The inability to de-duplicate records could lead to an exaggeration of the balance sheet during aggregation of the valuation field. Fache-Rousova and others (2016) in dealing with this produces a range of low, middle and high estimates, assigning 0%, 50% and 100% weight respectively to the unpaired trade between EU counterparties.
In this article (for example in Tables 2 and 3) we have assumed 100% weight as a rule of thumb. In other words, we are treating every unpaired inter-EU record as not duplicated elsewhere on the database. This is likely to result in an over-estimate, but equally, we think using a 50% weight will result in under-estimate, as it instead assumes that every unpaired inter-EU trade can be paired on the database. In reality the optimal weight is likely somewhere in between 50% and 100%.
We expect that some unpaired trades could have been matched if the use of trade IDs is perfect, but even then because of the cost barrier and likely under-compliance mentioned previously, it is unlikely that all unpaired trades would have been matched.
Treatment of cleared OTC trades
Under EMIR, a cleared OTC trade can be reported four times on the trade repositories database: two reports on the first leg of the trade between a clearing member and the central clearing counterparty (CCP), and two reports on the second leg between the CCP and the other clearing member, and the two legs would be recorded under different trade identifiers.
If the clearing members are trading on behalf of their clients, this can lead to even more reports and trade identifiers of the same trade being submitted to TRs. This feature of the EMIR data presents both theoretical and practical issues when we attempt to estimate the sectoral or economy-wide balance sheet.
The latest EU manual on compiling national accounts, the European System of Accounts 2010 (ESA 2010), is silent on the theoretical treatment of the clearing activities of CCP. The industry norm recognises a cleared OTC trade as one contract rather than multiple ones as reported under EMIR. On the other hand, it is also clear that a clearing arrangement involving a CCP disconnects the financial claim or obligation relationship that would have otherwise existed directly between the trading counterparties, and centralises these entitlements or obligations within the CCP.
For example, if a clearing member is unable to honour its cleared contracts and becomes bankrupt, the CCP through which these contracts are cleared is still obliged to pay the other trading party. Since a financial asset (liability) in ESA 2010 is defined as a financial claim (obligation) and since a clearing arrangement would by design create an extra layer of claim and obligation, there is an argument for the two legs of OTC-cleared trades to be counted as separate assets or liabilities from a national accounting point of view.
Tables 2 and 3 assume at the moment that the different legs will be counted as separate contracts. We understand that this could overstate the cleared OTC trades balance sheet in the economy compared with industry norm or even current data sources (which are not fully capturing CCP activities). The Office for National Statistics (ONS) will undertake an internal review to recommend on the appropriate treatment of CCP activities, and improve coverage of CCP activities in the Financial Services Survey.
Even if the theoretical ambiguity over the treatment of cleared OTC trades is resolved, there are still practical issues anyway in aggregating the data appropriately. In the event the internal review recommends treating the multiple reports over different legs as one contract, the lack of a common trade identifier linking the different legs of the same trade means that our ability to de-duplicate properly is severely limited and may have to rely on probabilistic matching approaches.
If the internal review recommends the different legs are to be treated separately, during the de-duplication process we can specify for the same trade identifier to use the CCP (both UK and non-UK) report and discard the clearing member report. This is the enhanced approach that we have implemented in producing Table 3, but it is not without caveats: there is an inconsistency issue in how OTC cleared trades are reported in the EMIR data.
If each cleared OTC trade is indeed reported four times (two times on each leg, one by the CCP and one by the clearing member), we expect the overall number of CCP reports and clearing member reports of cleared OTC trades to be similar across the entire EMIR data. We have found this to be untrue in the data and that there are many more CCP reports than clearing member reports (the difference stands at about 1 million). We are unable to ascertain exactly how much of the difference is driven by under-compliance by clearing members, over-reporting by CCP, or that some cleared trades are reported differently (and incorrectly) under other arrangements. These scenarios can have different implications on the robustness of balance sheet estimates of cleared OTC trades based purely on CCP reports.
Incorrect data
The EMIR reporting landscape has some unfavourable features that could increase the likelihood of incorrect data being submitted.
Firstly, counterparties self-report their activities and the onus is on them (or their delegates) to report diligently and correctly. However, as discussed previously, EMIR reporting is complicated and often does not speak directly to the recording databases of firms.
From speaking to a trade repository, there is evidence in the past that the EMIR field on valuation, which is supposedly the marked-to-market or marked-to-model valuation of the contract, had been interpreted as other measures1 simply because valuation is understood to be something else on the systems of individual firms.
The latest technical standards implemented at the end of 2017 now provide clearer definitions on valuation as well as other fields, but it remains the case that while firms may try to diligently input the data according to what they think is correct, the data may turn out to be wrong (such as in the wrong unit or currency). The enhanced data cleaning procedure, which filters the raw data based on the credibility of the reporters under different trading arrangements, mitigates this issue to a certain extent.
Secondly, the valuation field is not required for all contracts. In the Quarter 1 (Jan to Mar) 2017 data, after the enhanced cleaning procedure, we find that 32% of the 16 million open trade positions did not include a valuation. This can partly be explained by the EMIR requirement that only financial corporations and large non-financial corporations need to provide valuations. There could also be an element of under-compliance. Currently, in our methodology in compiling Tables 2 and 3 we do not attempt to impute the valuations but instead assign them as zero.
Thirdly, trade repositories only carry out limited validation of the data. They do not, and in most cases cannot, verify if the valuation field is corrected inputted.
Part of the issue is that valuation, which is crucial for national accounting, is only one of the over 100 fields in the data. As it is not mandatory, it is not validated actively. Where validation checks are run to reconcile paired reports on the same trade repository or across different repositories, they focus mostly on reconciling trade ID and the two counterparty IDs. This casts considerable doubt on the validity of the valuation field even when it is provided.
For example, we have seen cases where paired records can return significantly different valuation estimates, and in some cases they do not even agree on the sign, which directly affects the assignment of the contract as an asset or liability to a counterparty. Results from comparing EMIR and DQ estimates of the balance sheets of major banks show that the differences at the micro level, even after an outlier removal procedure, can be well over a trillion GBP apart. It is impossible that a bank’s derivative balance sheet can run to that magnitude, even if all its worldwide operations are counted and the coverage issue is taken into account. This shows that even after removing the most obvious outliers there are still significant issues with the quality of information held in the valuation field in the EMIR data. The enhanced data cleaning procedure again mitigates this issue to a certain extent.
Fourthly, the use of unique product identifier in EMIR through Classification of Financial Instrument (CFI) codes is uneven and it is reportedly an unpopular standard with the industry. Derivative contracts can be highly complex and it has been suggested that some products can fit more than one code, which can lead to inaccurate national accounts balance sheet estimates at product level if the enhanced de-duplication process is going to keep just of the two records that disagree on CFI code.
All that said, counterparty reporting using Legal Entity Identifier (LEI) number is a relative strength of the data, especially after the recent upgrade of the reporting technical standard, where very few reports now have an invalid or empty entry. This is largely because LEI is a field that trade repositories run validation checks on and can reject on the basis of.
Notes for: Annex C: Raw EMIR data quality concerns
- For example, some firms viewed valuation as “delta”, which is a measure of the impact on the price of an option of a change in the price of the underlying instrument.
13. Annex D: Alternative data sources for derivatives and their comparison to EMIR
We discuss and compare with the European Market Infrastructure Regulation (EMIR) data the properties of the following alternative data sources for information on derivatives trade: Bank of England’s Derivative Quarterly (DQ) Survey, the ONS’s Financial Services Survey (FSS), the ONS’s Trusts Survey, the Solvency II (SII) administrative data on insurance companies, the ONS’s Pension Funds Survey (PFS), the ONS’s Trusts Surveys and the market in financial instruments directive II (MiFID II) administrative data.
Derivatives Quarterly (DQ) Survey
The DQ Survey is conducted by the Bank of England. It goes out every quarter to approximately 20 major banks in the UK (some of which are UK branches of foreign banks), asking for their end-of-quarter asset and liability positions in different derivative contracts against different sectors of the economy.
It is designed for UK National Accounts purposes and is the current data source for derivative balance sheet of the S.122 deposit-taking corporations except central banks subsector (which makes up the majority of the derivatives balance sheet of the MFI subsector). In terms of coverage, granularity, quality and availability of from-whom-to-whom (FWTW) information, the DQ Survey can be considered as a strong dataset.
The DQ reporting population account for about 95% of the S.122 subsector’s derivatives balance sheet1. Since DQ is designed for national accounts purposes, the concepts behind the survey are fully aligned with national accounts requirements. For example, it asks reporters to only report exposures of their UK resident offices. The survey also covers UK branches of foreign incorporated banks. This is in contrast to EMIR reporting, where the reports of a UK incorporated legal entity may also include the activity of foreign branches, and non-EU entities are not required to report the local activities of their foreign branches (including those located in the UK).
When returning the form, reporters are asked to provide a breakdown of their derivative asset and liability position across three dimensions: the underlying asset class, contract type and the contract currency.
The asset class dimension contains four categories: interest rate, foreign exchange, credit derivative, and other.
The contract type dimension contains five categories: swaps, options, forward rate agreements, futures/forwards, and other.
The currency dimension contains three categories: euro, pound sterling, and other currencies.
In terms of FWTW information, the form asks for positions against 13 counterparty sector categories. The interaction of instrument and counterparty breakdown means that DQ data contain highly granular information on the composition of the banks’ derivative asset and liability positions.
While the DQ Survey has a very small sample compared with a large administrative dataset like the EMIR data, a clear advantage is that the quality of the data is likely to be much higher as it is more focused, since it is more feasible to provide support to respondents and apply close scrutiny to submissions.
Overall, we consider the DQ Survey a better data source than the EMIR data in estimating the derivatives balance sheet and FWTW positions of the S.122 subsector. It already contains a good level of granularity in instrument and counterparty sector breakdown, but there is still scope for the EMIR data to provide extra information in breaking down the “others” asset class, contract type and currencies.
Financial Services Survey (FSS)
The FSS is run by the Office for National Statistics (ONS) and the Securities Dealers Survey within it is the current data source for the OFI subsector’s derivatives balance sheet estimates. The FSS is identified as the main data source under the enhanced financial accounts initiative to improve coverage for the “shadow banking” sector (see Miller (2018)). The FSS sample covers 20 of the 21 Standard Industrial Classification (SIC) codes that map approximately to the financial subsectors of other financial intermediaries, (S.125), financial auxiliaries (S.126) and captive financial institutions and money lenders (S.127).
The survey goes out quarterly to a sample of about 2,000 firms. There are eight questions in the FSS form 266 that cover derivative positions.
The first four are answered by all respondents and cover the overall asset and liability position and a simple option/forward and local banks/local non-banks/RoW breakdown.
The other four questions in a modified template cover more detailed counterparty breakdown, but are only answered by firms from two SIC industries: security dealing on own account (64.991, mapped to S.125) and security and commodity contracts brokerage (66.12, mapped to S.126). The reason is that the firms in these industries, especially the security dealers, are thought to account for most of the derivative assets and liabilities of all FSS respondents.
In this sense, the FSS can be considered a source of granular information on the counterparty sector breakdown of the derivative contracts held in the subsectors S.125 to S.127. However, at the same time improving the coverage of the FSS over CCP activities (S.125) has been identified as a future area of ONS work (see Miller 2018). This raises concern for using the FSS to estimate the derivatives balance sheet of subsector S.125 as CCPs are major players in the OTC market.
Indeed, the EMIR data suggest that within the financial subsectors of S.125, S.126 and S.127, contracts held by firms from industries 66.11 (administration of financial markets) and 66.19 (other activities) can account for over 80% of the balance sheet. This paints a different picture to what the FSS currently tells us. Ongoing work at the ONS is looking to refresh the FSS sample to address such potential issues.
On the availability of granular information on derivative instrument breakdown, as mentioned already the FSS only asks for the positions by options and forward-type contracts. Unlike form DQ (derivative quarterly), it does not distinguish contracts by the underlying asset or the currency of denomination. The ongoing internal review on the FSS may address this.
The quality of information collected via the FSS can be considered as good. The returns are subject to the standard validation routine at the ONS. Where there are big swings in the firms’ returns between quarters, there is available resource to get in touch with them to understand if the movements are genuine or if there have been mistakes.
Overall, we consider the FSS a better data source than the EMIR data in estimating the sectoral derivatives balance sheet and FWTW positions for the S.125 to S.127 subsectors, particularly once the sampling framework is adjusted to cover all central clearing counterparties. On the other hand, there is scope for the EMIR data to inform more granular instrument breakdown of the derivative assets and liabilities held by these subsectors.
Solvency II (SII) administrative data
The Solvency II directive on harmonising the EU insurance regulation has led to a rich administrative dataset on the quarterly balance sheet of insurance corporations, on the basis of which the ONS has produced experimental statistics for the balance sheet of the insurance corporations (S.128) sector (see Akritidis (2018)).
The coverage of the data over the UK insurance corporations subsector is not perfect but not far from it. In a population of around 400 insurance firms, about 180 smaller companies are eligible for a waiver and do not return data for all periods or only return certain templates. However, as most of the activities are undertaken by large companies, the impact of the waivers on aggregates is likely small. The SII data cover 97% of UK insurers’ economic activity.
There are two Solvency II reporting templates that contain detailed questions on derivative contracts held by the reporting insurance corporations. One is on open positions and the other one is on transactions. In theory, the two templates can be used to estimate the stocks and flows of derivative assets of the S.128 subsector.
Similar to EMIR, under Solvency II each derivative contract shall be reported separately. Aggregated or net reporting is only allowed for frequent trades on the same derivative if all the relevant characteristics of the contracts are common. The data include all the necessary fields for compiling FWTW estimates in the flow of funds account: counterparty identifier (both name and LEI where possible), product identifier (in ISIN code where available), valuation and currency of contract or valuation.
The quality of the SII data has been subject to ONS scrutiny prior to the production of the experimental statistics on the insurance corporations subsector, and hence can be considered as good. For example, Akritidis (2018) shows that the sectoral net position in derivatives of the insurance incorporations subsector is close to zero, which is a signal of the quality of the data.
Overall, we consider the SII data a better data source than the EMIR data in estimating the sectoral derivatives balance sheet and FWTW positions for the S.128 subsector. There may be scope for the EMIR to supplement additional breakdown such as collateralisation if there is user demand.
Pension Funds Survey (PFS)
The ONS Pension Funds Survey, published as part of the MQ5 statistical bulletin, goes out to approximately 360 UK-based pension funds. It collects information about the derivatives balance sheet in the annual survey, and flows and transactions in the quarterly survey.
The granularity of information on both counterparty and instruments breakdown is limited. There is a UK versus overseas split, and in the annual survey, balance sheet information is asked for five contract types: credit default products, other options, other swaps, futures and forwards, and other.
The quality of information collected via the PFS can be considered as good. The returns are subject to the standard validation routine at the ONS. Where there are big swings in the firms’ returns between periods, there is available resource to get in touch with them to understand if the movements are genuine or if there have been mistakes.
From Quarter 2 (Apr to June) 2019 onwards, the PFS in its current form will be replaced by a new online survey. It will include quarterly derivative balance sheet estimates with a four-instrument breakdown: swaps, options, forward rate agreements, and futures, and a simple UK versus overseas split.
Overall, we consider this survey a better data source to the EMIR data in estimating the sectoral derivative balance sheet for the S.129 pension funds subsector. On the other hand, there is scope for the EMIR data to inform more granular counterparty sector and instrument breakdown of the derivative assets and liabilities held by pension funds.
Trusts Surveys
The ONS Trusts Surveys are the current data sources for estimating the balance sheet of the subsector S.124 non-money market funds investment trust (NMMFs). They compose of three separate surveys: on investment trusts, unit trusts and property unit trusts.
They are quarterly and annual surveys that sample investment funds managers rather the funds that they manage, so they happen to capture some money market funds that belong to subsector S.123 money market funds (MMFs). The exact split of assets and liabilities by MMFs and NMMFs is therefore not known within the surveys and the national accounts, though experimental results from the FoF initiative currently estimate that the size of MMFs is about 2% of NMMFs. Coverage of the surveys is good over traditional investment funds, but it is limited in regards to non-traditional investment funds that are becoming more important, such as hedge funds, private equity, exchange-traded funds, and so on.
Available granularity in counterparties and derivative type is limited in the Trusts Survey. In the annual balance sheet surveys there are breakdowns of derivative assets and liabilities by holdings against UK monetary financial institutions (MFIs), other UK and rest of the world (RoW). There is no breakdown of derivative asset class or contract type in the Balance Sheet Survey. In the Quarterly Transactions Survey, there are counterparty breakdowns of derivative contracts by UK and overseas, and instrument breakdowns by interest rate swaps, forward rate agreement and other derivative contracts.
As part of the flow of funds (FoF) initiative the ONS will be reviewing the Trusts Surveys to consider how to improve coverage of non-traditional investment funds. Since the Trusts Surveys are considered a strong data source for traditional investment fund types and that some of the missing coverage may not be relevant for the UK (see Miller (2018) for a discussion on the domicile of hedge funds), we judge that they are a better data source than the EMIR data in estimating the sectoral derivatives balance sheet of subsector S.124.
On the other hand, there is scope for the EMIR data to inform more granular counterparty and instrument breakdown. As for subsector S.123, the Trusts Surveys may not provide a good solution. Instead, the DQ and FSS could provide estimates for some of the main counterparty cells involving S.123, and the EMIR data could be used to estimate the rest.
Market in Financial Instruments Directive II (MiFID II) administrative data
The Markets in Financial Instruments Directive (MiFID) is a set of EU regulation that regulates firms who provide services to clients linked to financial instruments and the venues where those instruments are traded. It applied in the UK from November 2007 and was revised by MiFID II in January 2018. Data on transactions of instruments and open positions are collected at the entity level as part of the directive.
While the MiFID II datasets are designed for detecting market abuse and not for national accounting, many of their features are in general useful for compiling the national accounts. For example, data coverage is based on instruments listed or traded at venue and so is aligned with what the national accounts are trying to measure. Counterparty information on transactions is mostly available. The data quality is good because the FCA in compiling the data has strong quality control in place, with about 80 business logic rules plus further pattern checks that are all harmonised across Europe. The FCA also has the power to, and has in the past, penalised firms for under-compliance.
There are two MiFID II datasets that contain information on derivatives: the commodity positions and the transaction reports. Unfortunately, both datasets have features that make them unfitting for compiling derivatives FoF estimates: the commodity positions data contain no counterparty information, while the transaction reports contain no valuation of the contracts. The lack of these features is a reflection that the MiFID II data are collected for different purposes to national accounting.
We consider the MiFID II unsuitable for compiling derivative statistics in the national accounts. That said, it is likely that for some other types of financial instruments MiFID II can be a valuable data source to the enhanced financial accounts initiative.
Notes for: Annex D: Alternative data sources for derivatives and their comparison to EMIR
- The Bank of England verifies this by using data on total derivative positions collected via form BT (balance sheet), which all deposit-taking corporations in the UK are required to complete.